|
- Kyung Won Kim, Jimi Huh, Bushra Urooj, Jeongjin Lee, Jinseok Lee, In-Seob Lee, Hyesun Park, Seongwon Na, Yousun Ko, Artificial Intelligence in Gastric Cancer Imaging With Emphasis on Diagnostic Imaging and Body Morphometry, Journal of Gastric Cancer, Vol. 23, No. 3, pp. 388-399, July 2023. (doi:10.5230/jgc.2023.23.e30)
- Heeryeol Jeong, Taeyong Park, Seungwoo Khang, Kyoyeong Koo, Juneseuk Shin, Kyung Won Kim, Jeongjin Lee (Corresponding author), Non-rigid Registration Based on Hierarchical Deformation of Coronary Arteries in CCTA Images, Biomedical Engineering Letters, Vol. 13, No. 1, pp. 65-72, February 2023. (doi:10.1007/s13534-022-00254-8)
 |
Objective: In this paper, we propose an accurate and rapid non-rigid registration method between blood vessels in temporal 3D cardiac computed tomography angiography images of the same patient. This method provides auxiliary information that can be utilized in the diagnosis and treatment of coronary artery diseases. Methods: The proposed method consists of the following four steps. First, global registration is conducted through rigid registration between the 3D vessel centerlines obtained from temporal 3D cardiac CT angiography images. Second, point matching between the 3D vessel centerlines in the rigid registration results is performed, and the corresponding points are defined. Third, the outliers in the matched corresponding points are removed by using various information such as thickness and gradient of the vessels. Finally, non-rigid registration is conducted for hierarchical local transformation using an energy function. Results: The experiment results show that the average registration error of the proposed method is 0.987 mm, and the average execution time is 2.137 s, indicating that the registration is accurate and rapid. Conclusion: The proposed method that enables rapid and accurate registration by using the information on blood vessel characteristics in temporal CTA images of the same patient.
|
- Seungwoo Khang, Taeyong Park, Junwoo Lee, Kyung Won Kim, Hyunjoo Song, Jeongjin Lee, Computer-Aided Breast Surgery Framework Using a Marker-less Augmented Reality Method, Diagnostics, Vol. 12, No. 12:3123, pp. 1-13, December 2022. (doi:10.3390/diagnostics12123123)
- Sunyoung Lee, Kyoung Won Kim, Heon-Ju Kwon, Jeongjin Lee, Gi-Won Song, Sung-Gyu Lee, Impact of the preoperative skeletal muscle index on early remnant liver regeneration in living donors after liver transplantation, Korean Journal of Transplantation, Vol. 36, No. 4, pp. 259-266, December 2022. (doi:10.4285/kjt.22.0039)
- Dong Wook Kim, Hyemin Ahn, Kyung Won Kim, Seung Soo Lee, Hwa Jung Kim, Yousun Ko, Taeyong Park, Jeongjin Lee, Jiyeon Ha, Hyemin Ahn, Yu Sub Sung, Hong-Kyu Kim, Prognostic Value of Sarcopenia and Myosteatosis in Patients with Resectable Pancreatic Ductal Adenocarcinoma, Korean Journal of Radiology, Vol. 23, No. 11, pp. 1055-1066, November 2022. (doi:10.3348/kjr.2022.0277)
- Heon-Ju Kwon, Kyoung Won Kim, Kyung A Kang, Mi Sung Kim, So Yeon Kim, Taeyong Park, Jeongjin Lee, Oral effervescent agent improving magnetic resonance cholangiopancreatography, Quantitative Imaging in Medicine and Surgery, Vol. 12, No. 9, pp. 4414-4423, September 2022. (doi:10.21037/qims-22-219)
- Sunyoung Lee, Kyoung Won Kim, Jeongjin Lee, Sex-specific Cutoff Values of Visceral Fat Area for Lean vs Overweight/obese Nonalcoholic Fatty Liver Disease in Asians, Journal of Clinical and Translational Hepatology, Vol. 10, No. 4, pp. 595-599, August 2022. (doi:10.14218/JCTH.2021.00379)
- Sun Hong, Kyung Won Kim, Hyo Jung Park, Yousun Ko, Changhoon Yoo, Seo Young Park, Seungwoo Khang, Heeryeol Jeong, Jeongjin Lee, Impact of Baseline Muscle Mass and Myosteatosis on Early Toxicity during First-line Chemotherapy for Initially Metastatic Pancreatic Cancer, Frontiers in Oncology, Vol. 12, Article Number. 878472, May 2022. (doi:10.3389/fonc.2022.878472)
- Taeyong Park, Min A Yoon, Young Chul Cho, Su Jung Ham, Yousun Ko, Sehee Kim, Heeryeol Jeong, Jeongjin Lee, Automated Segmentation of the Fractured Vertebrae on CT and Its Applicability in a Radiomics Model to Predict Fracture Malignancy, Scientific Reports, Vol. 12, Article Number. 6735, April 2022. (doi:10.1038/s41598-022-10807-7)
- Yousun Ko, Heeryoel Jeong, Seungwoo Khang, Jeongjin Lee, Kyung Won Kim, Beom-Jun Kim, Change of Computed Tomography-based Body Composition after Adrenalectomy in Patients with Pheochromocytoma, Cancers, Vol. 14, No. 1967, pp. 1-12, April 2022. (doi:10.3390/cancers14081967)
- Sunyoung Lee, Kyoung Won Kim, Heon-Ju Kwon, Jeongjin Lee, Kyoyeong Koo, Gi-Won Song, Sung-Gyu Lee, Relationship of Body Mass Index and Abdominal Fat with Radiation Dose Received during Preoperative Liver CT in Potential Living Liver Donors: a Cross-sectional Study, Quantitative Imaging in Medicine and Surgery, Vol. 12, No. 4, pp. 2206-2212, April 2022. (doi:10.21037/qims-21-977)
- Sunyoung Lee, Kyoung Won Kim, Jeongjin Lee, Taeyong Park, Kyoyeong Koo, Gi-Won Song, Sung-Gyu Lee, Visceral Fat Area is an Independent Risk Factor for Overweight or Obese Nonalcoholic Fatty Liver Disease in Potential Living Liver Donors, Transplantation Proceedings, Volume 54, Issue 3, pp. 702-705, April 2022. (doi:10.1016/j.transproceed.2021.10.032)
- Taeyong Park, Seungwoo Khang, Heeryeol Jeong, Kyoyeong Koo, Jeongjin Lee, Juneseuk Shin, Ho Chul Kang, Deep Learning Segmentation in 2D X-ray Images and Non-rigid Registration in Multi-modality Images of Coronary Arteries, Diagnostics, Vol. 12, No. 4:778, pp. 1-21, March 2022. (doi:10.3390/diagnostics12040778)
- Jiyeon Ha, Taeyong Park, Hong-Kyu Kim, Youngbin Shin, Yousun Ko, Dong Wook Kim, Yu Sub Sung, Jiwoo Lee, Su Jung Ham, Seungwoo Khang, Heeryeol Jeong, Kyoyeong Koo, Jeongjin Lee, Kyung Won Kim, Development of a Fully Automatic Deep Learning System for L3 Selection and Body Composition Assessment on Computed Tomography, Scientific Reports, Vol. 11, Article Number. 21656, November 2021. (doi:10.1038/s41598-021-00161-5)
- Dong Wook Kim, Kyung Won Kim, Yousun Ko, Taeyong Park, Jeongjin Lee, Jiyeon Ha, Hyemin Ahn, Yu Sub Sung, Hong-Kyu Kim, Effects of the Contrast Phase on Computed Tomography Measurements of Muscle Quantity and Quality, Korean Journal of Radiology, Vol. 22, No. 11, pp. 1909-1917, November 2021. (doi:10.3348/kjr.2021.0105)
- Sunyoung Lee, Kyoung Won Kim, Jeongjin Lee, Taeyong Park, Seungwoo Khang, Heeryeol Jeong, Gi-Won Song, Sung-Gyu Lee, Visceral Adiposity as a Risk Factor for Lean Nonalcoholic Fatty Liver Disease in Potential Living Liver Donors, Journal of Gastroenterology and Hepatology, Vol. 36, Issue 11, pp. 3212-3218, November 2021. (doi:10.1111/jgh.15597)
- Sunyoung Lee, Kyoung Won Kim, Jeongjin Lee, Taeyong Park, Hyo Jung Park, Gi-Won Song, Sung-Gyu Lee, Reduction of Visceral Adiposity as a Predictor for Resolution of Nonalcoholic Fatty Liver in Potential Living Liver Donors, Liver Transplantation, Vol. 27, Issue 10, pp. 1424-1431, October 2021. (doi:10.1002/lt.26071)
- Hyo Jung Park, Kyoung Won Kim, Jeongjin Lee, Taeyong Park, Heon-Ju Kwon, Gi-Won Song, Sung-Gyu Lee, Change in hepatic volume profile in potential live liver donors after lifestyle modification for reduction of hepatic steatosis, Abdominal Radiology, Vol. 46, No. 8, pp. 3877-3888, August 2021. (doi:10.1007/s00261-021-03058-z)
- Ja Kyung Yoon, Sunyoung Lee, Kyoung Won Kim, Ji Eun Lee, Jeong Ah Hwang, Taeyong Park, Jeongjin Lee, Reference Values for Skeletal Muscle Mass at the Third Lumbar Vertebral Level Measured by Computed Tomography in a Healthy Korean Population, Endocrinology and Metabolism, Vol. 36, No. 3, pp. 672-677, June 2021. (doi:10.3803/EnM.2021.1041).
- So Yeong Jeong, Kyoung Won Kim, Jeongjin Lee, Jin Kyoo Jang, Heon-Ju Kwon, Gi Won Song, Sung Gyu Lee, Hepatic volume profiles in potential living liver donors with anomalous right-sided ligamentum teres, Abdominal Radiology, Vol. 46, No. 4, pp. 1562-1571, April 2021. (doi:10.1007/s00261-020-02803-0)
- Dong Wook Kim, Jiyeon Ha, Yousun Ko, Kyung Won Kim, Taeyong Park, Jeongjin Lee, Myung-Won You, Kwon-Ha Yoon, Ji Yong Park, Young Jin Kee, Hong-Kyu Kim, Reliability of Skeletal Muscle Area Measurement on CT with Different Parameters: a Phantom Study, Korean Journal of Radiology, Vol. 22, No. 4, pp. 624-633, April 2021. (doi:10.3348/kjr.2020.0914)
- Minyoung Chung, Jingyu Lee, Sanguk Park, Chae Eun Lee, Jeongjin Lee (Corresponding author), Yeong-Gil Shin, Liver Segmentation in Abdominal CT Images via Auto-Context Neural Network and Self-Supervised Contour Attention, Artificial Intelligence in Medicine, Vol. 113, Article 102023, March 2021. (doi:10.1016/j.artmed.2020.101996) (IF=4.383, JCR 2019)
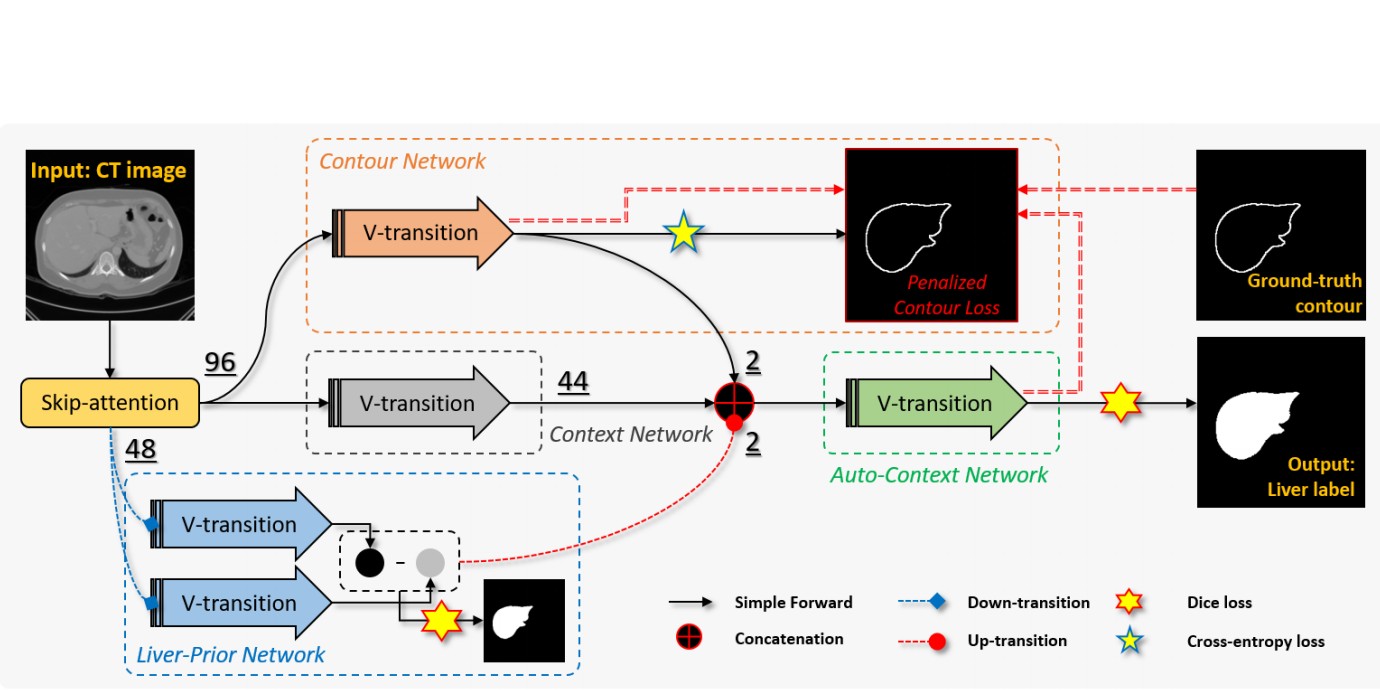 |
Objective: Accurate image segmentation of the liver is a challenging problem owing to its large shape variability and unclear boundaries. Although the applications of fully convolutional neural networks (CNNs) have shown groundbreaking results, limited studies have focused on the performance of generalization. In this study, we introduce a CNN for liver segmentation on abdominal computed tomography (CT) images that focus on the performance of generalization and accuracy. Methods: To improve the generalization performance, we initially propose an auto-context algorithm in a single CNN. The proposed auto-context neural network exploits an effective high-level residual estimation to obtain the shape prior. Identical dual paths are effectively trained to represent mutual complementary features for an accurate posterior analysis of a liver. Further, we extend our network by employing a self-supervised contour scheme. We trained sparse contour features by penalizing the ground-truth contour to focus more contour attentions on the failures. Results: We used 180 abdominal CT images for training and validation. Two-fold cross-validation is presented for a comparison with the state-of-the-art neural networks. The experimental results show that the proposed network results in better accuracy when compared to the state-of-the-art networks by reducing 10.31% of the Hausdorff distance. Novel multiple N-fold cross-validations are conducted to show the best performance of generalization of the proposed network. Conclusion and Significance: The proposed method minimized the error between training and test images more than any other modern neural networks. Moreover, the contour scheme was successfully employed in the network by introducing a self-supervising metric. |
- Donggeon Oh, Bohyoung Kim, Jeongjin Lee (Corresponding author), Yeong-Gil Shin, Unsupervised Deep Learning Network with Self-attention Mechanism for Non-rigid Registration of 3D Brain MR Images, Journal of Medical Imaging and Health Informatics, Vol. 11, No. 3, pp. 736-751, March 2021. (doi:10.1166/jmihi.2020.3345)
 
|
In non-rigid registration for medical imaging analysis, computation is complicated, and the high accuracy and robustness needed for registration are difficult to obtain. Recently, many studies have been conducted for non-rigid registration via unsupervised learning networks. This study proposes a method to improve the performance of this unsupervised learning network approach, through the use of a self-attention mechanism. In this paper, the self-attention mechanism is combined with deep learning networks to identify information of higher importance, among large amounts of data, and thereby solve specific tasks. Furthermore, the proposed method extracts both local and non-local information so that the network can create feature vectors with more information. As a result, the limitation of the existing network is addressed: alignment based solely on the entire silhouette of the brain is mitigated in favor of a network which also learns to perform registration of the parts of the brain that have internal structural characteristics. To the best of our knowledge, this is the first such utilization of the attention mechanism in this unsupervised learning network for non-rigid registration. The proposed attention network performs registration that takes into account the overall characteristics of the data, thus yielding more accurate matching results than those of the existing methods. In particular, matching is achieved with especially high accuracy in the gray matter and cortical ventricle areas, since these areas contain many of the structural features of the brain. The experiment was performed on 3D magnetic resonance images of the brains of 50 people. The measured average dice similarity coefficient after registration was 70.40%, which is an improvement of 17.48% compared to that before registration. This improvement indicates that application of the attention block can further improve the performance by an additional 8.5%, as relative to that without attention block. Ultimately, through implementation of non-rigid registration via the attention block method, the internal structure and overall shape of the brain can be addressed, without additional data input. Additionally, attention blocks have the advantage of being able to easily connect to existing networks without a significant computational overhead. Furthermore, by producing an attention map, the area of the brain around which registration was more performed can be visualized. This approach can be used for non-rigid registration with various types of medical imaging data. |
- Jiwan Kim, Jeongjin Lee, Minyoung Chung, Yeong-Gil Shin, Multiple weld seam extraction from RGB-depth images for automatic robotic welding via point cloud registration, Multimedia Tools and Applications, Volume 80, Issue 6, pp. 9703-9719, March 2021. (doi:10.1007/s11042-020-10138-7)
- Taeyong Park, Jeongjin Lee, Juneseuk Shin, Kyoung Won Kim, Ho Chul Kang, Non-rigid liver registration in liver computed tomography images using elastic method with global and local deformations, Journal of Medical Imaging and Health Informatics, Vol. 11, No. 3, pp. 810-816, March 2021. (doi:10.1166/jmihi.2020.3355)
- Dongjoon Kim, Heewon Kye, Jeongjin Lee (Corresponding author), Yeong-Gil Shin, Confidence-controlled Local Isosurfacing, IEEE Transactions on Visualization and Computer Graphics, Vol. 27, No. 1, pp. 29-42, January 2021. (doi:10.1109/TVCG.2020.3016327) (IF : 3.780, JCR 2019)
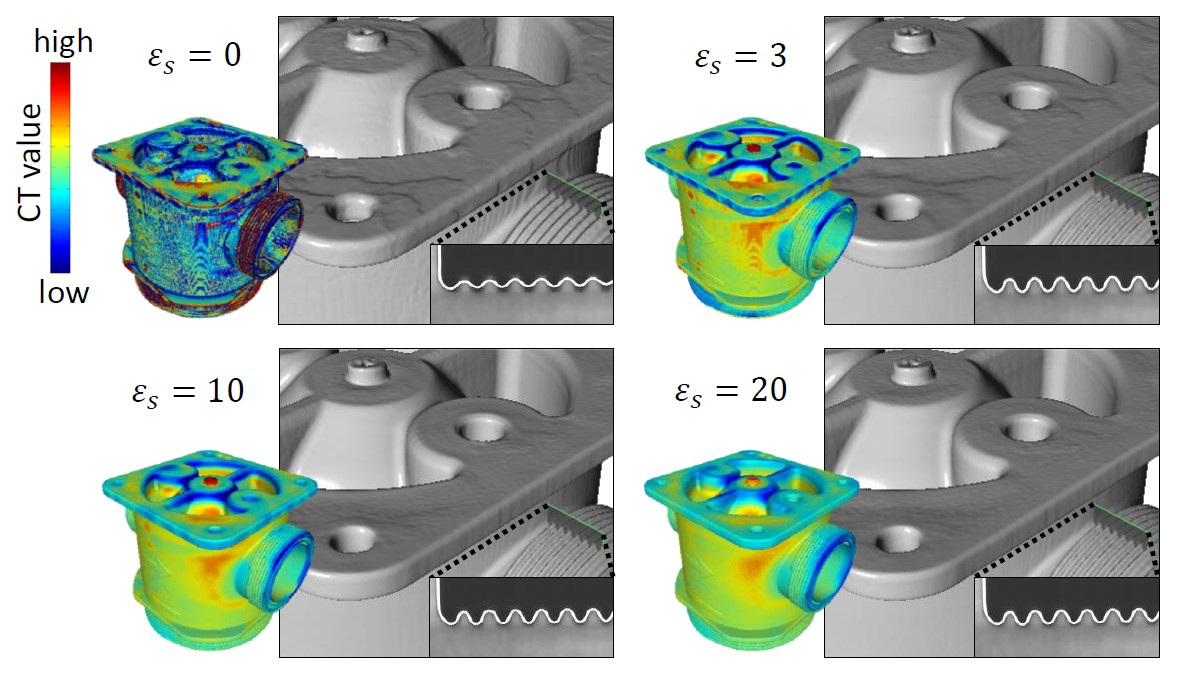 |
This paper presents a novel framework that can generate a high-fidelity isosurface model of X-ray computed tomography (CT) data. CT surfaces with subvoxel precision and smoothness can be simply modeled via isosurfacing, where a single CT value represents an isosurface. However, this inevitably results in geometric distortion of the CT data containing CT artifacts. An alternative is to treat this challenge as a segmentation problem. However, in general, segmentation techniques are not robust against noisy data and require heavy computation to handle the artifacts that occur in three-dimensional CT data. Furthermore, the surfaces generated from segmentation results may contain jagged, overly smooth, or distorted geometries. We present a novel local isosurfacing framework that
can address these issues simultaneously. The proposed framework exploits two primary techniques: 1) Canny edge approach for obtaining surface candidate boundary points and evaluating their confidence and 2) screened Poisson optimization for fitting a surface to the boundary points in which the confidence term is incorporated. This combination facilitates local isosurfacing that can produce high-fidelity surface models. We also implement an intuitive user interface to alleviate the burden of selecting the appropriate confidence computing parameters. Our experimental results demonstrate the effectiveness of the proposed framework. |
- Youngbin Shin, Jimi Huh, Su Jung Ham, Young Chul Cho, Yoonseok Choi, Dong-Cheol Woo, Jeongjin Lee, Kyung Won Kim, Test-retest repeatability of ultrasonographic shear wave elastography in a rat liver fibrosis model: toward a quantitative biomarker in a preclinical trial, Ultrasonography, Vol. 40, No. 1, pp. 126-135, January 2021. (doi:10.14366/usg.19088)
- Minyoung Chung, Jusang Lee, Sanguk Park, Minkyung Lee, Chae Eun Lee, Jeongjin Lee, Yeong-Gil Shin, Individual Tooth Detection and Identification from Dental Panoramic X-Ray Images via Point-wise Localization and Distance Regularization, Artificial Intelligence in Medicine, Vol. 111, Article 101996, January 2021. (doi:10.1016/j.artmed.2020.101996)(IF=4.383, JCR 2019).
- Minyoung Chung, Jingyu Lee, Wisoo Song, Youngchan Song, Il-Hyung Yang, Jeongjin Lee (Corresponding author), Yeong-Gil Shin, Automatic Registration between Dental Cone-Beam CT and Scanned Surface via Deep Pose Regression Neural Networks and Clustered Similarities, IEEE Transactions on Medical Imaging, Vol. 39, No. 12, pp. 3900-3909, December 2020. (doi:10.1109/TMI.2020.3007520) (IF : 6.685, JCR 2019)
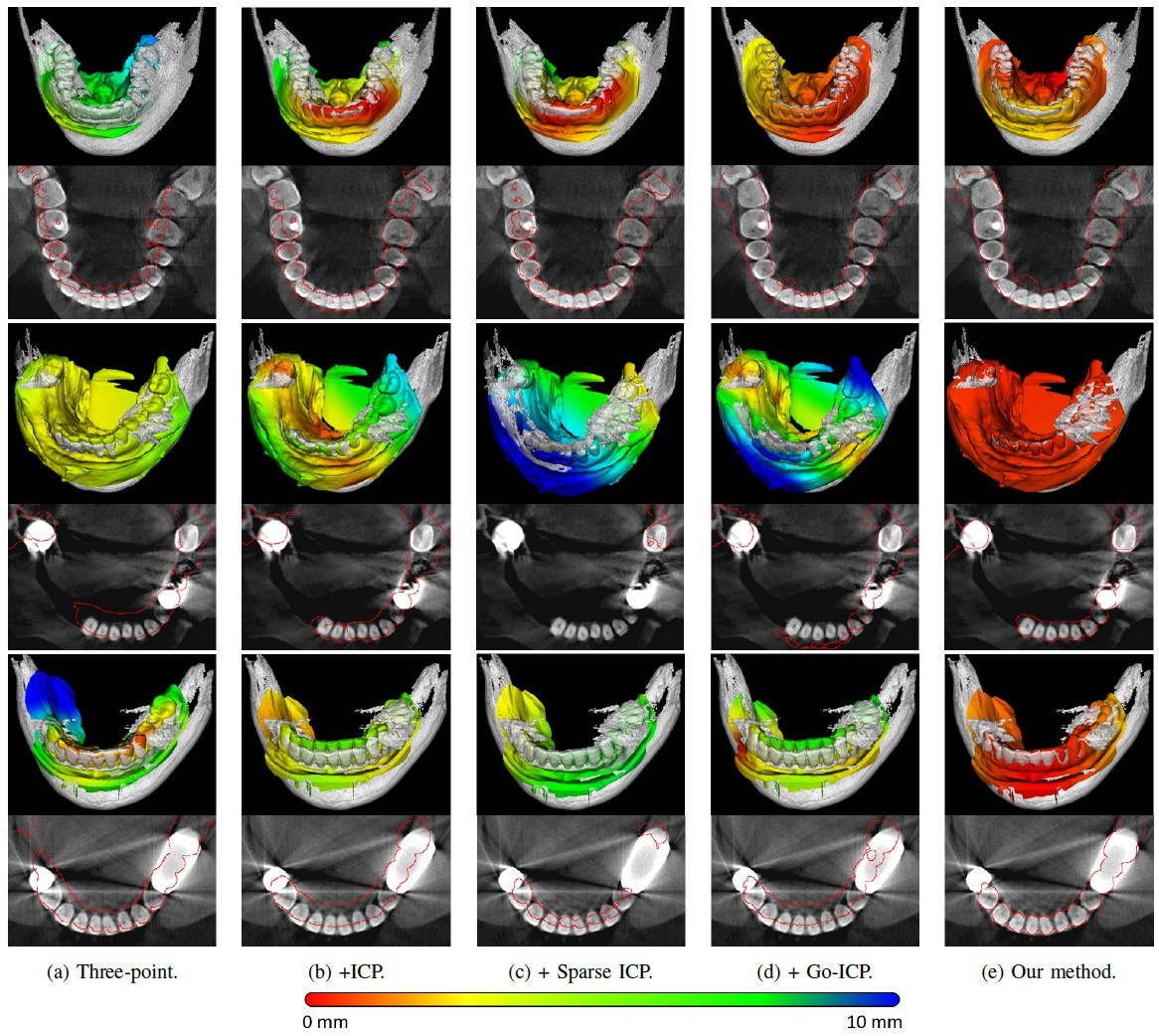 |
Computerized registration between maxillofacial cone-beam computed tomography (CT) images and a scanned dental model is an essential prerequisite for surgical planning for dental implants or orthognathic surgery. We propose a novel method that performs fully automatic registration between a cone-beam CT image and an optically scanned model. To build a robust and automatic initial registration method, deep pose regression neural networks are applied in a reduced domain (i.e., two-dimensional image). Subsequently, fine registration is performed using optimal clusters. A majority voting system achieves globally optimal transformations while each cluster attempts to optimize local transformation parameters. The coherency of clusters determines their candidacy for the optimal cluster set. The outlying regions in the iso-surface are effectively removed based on the consensus among the optimal clusters. The accuracy of registration is evaluated based on the Euclidean distance of 10 landmarks on a scanned model, which have been annotated by experts in the field. The experiments show that the registration accuracy of the proposed method, measured based on the landmark distance, outperforms the best performing existing method by 33.09%. In addition to achieving high accuracy, our proposed method neither requires human interactions nor priors (e.g., iso-surface extraction). The primary significance of our study is twofold: 1) the employment of lightweight neural networks, which indicates the applicability of neural networks in extracting pose cues that can be easily obtained and 2) the introduction of an optimal cluster-based registration method that can avoid metal artifacts during the matching procedures. |
- Jiseon Kang, Jeongjin Lee, Yeong-Gil Shin, Bohyoung Kim, Depth-of-Field Rendering using Progressive Lens Sampling in Direct Volume Rendering, IEEE Access, Vol. 8, Issue 1, pp. 93335-93345, December 2020. (doi:10.1109/ACCESS.2020.2994378) (IF : 3.745, JCR 2019)
- Dong Wook Kim, Kyung Won Kim, Yousun Ko, Taeyong Park, Seungwoo Khang, Heeryeol Jeong, Kyoyeong Koo, Jeongjin Lee, Hong-Kyu Kim, Jiyeon Ha, Yu Sub Sung, Youngbin Shin, Assessment of myosteatosis on computed tomography by automatic generation of muscle quality map using a web-based toolkit: Feasibility study, JMIR Medical Informatics, Vol. 8, Issue 8, e23049, pp. 1-8, October 2020. (doi:10.2196/23049)
- Heon-Ju Kwon, Kyoung Won Kim, Jong Keon Jang, Jeongjin Lee, Gi-Won Song, Sung-Gyu Lee, Reproducibility and reliability of CT volumetry in estimation of the right-lobe graft weight in adult-to-adult living donor liver transplantation: Cantlie¡¯s line vs. portal vein territorialization, Journal of Hepato-Biliary-Pancreatic Sciences, Volume 27, Issue 8, pp. 541-547, August 2020. (doi:10.1002/jhbp.749)
- Minyoung Chung, Jingyu Lee, Minkyung Lee, Jeongjin Lee (Corresponding author), Yeong-Gil Shin, Deeply Self-Supervised Contour Embedded Neural Network Applied to Liver Segmentation, Computer Methods and Programs in Biomedicine, Vol. 192, Article 105447, pp. 1-11, August 2020. (doi:10.1016/j.cmpb.2020.105447) (IF : 3.632, JCR 2019)
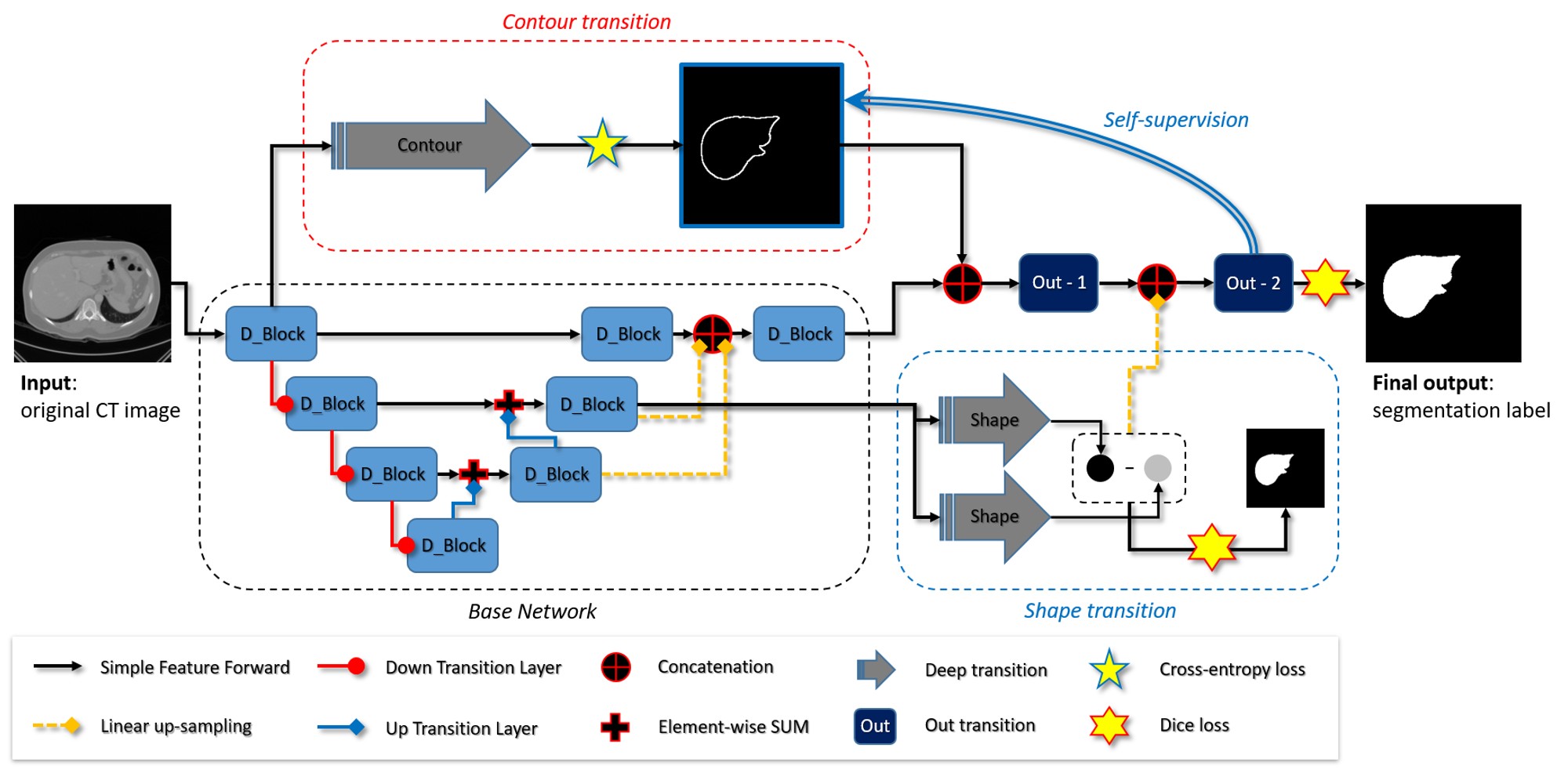 |
Objective: Herein, a neural network-based liver segmentation algorithm is proposed, and its performance was evaluated using abdominal computed tomography (CT) images. Methods: A fully convolutional network was developed to overcome the volumetric image segmentation problem. To guide a neural network to accurately delineate a target liver object, the network was deeply supervised by applying the adaptive self-supervision scheme to derive the essential contour, which acted as a complement with the global shape. The discriminative contour, shape, and deep features were internally merged for the segmentation results. Results and Conclusion: 160 abdominal CT images were used for training and validation. The quantitative evaluation of the proposed network was performed through an eight-fold cross-validation. The result showed that the method, which uses the contour feature, segmented the liver more accurately than the state-of-the-art with a 2.13% improvement in the dice score. Significance: In this study, a new framework was introduced to guide a neural network and learn complementary contour features. The proposed neural network demonstrates that the guided contour features can significantly improve the performance of the segmentation task. |
- Minyoung Chung, Minkyung Lee, Jioh Hong, Sanguk Park, Jusang Lee, Jingyu Lee, Il-Hyung Yang, Jeongjin Lee (Corresponding author), Yeong-Gil Shin, Pose-Aware Instance Segmentation Framework from Cone Beam CT Images for Tooth Segmentation, Computers in Biology and Medicine, Vol. 120, Article 103720, pp. 1-11, May 2020. (doi:10.1016/j.compbiomed.2020.103720) (IF : 3.434, JCR 2019)
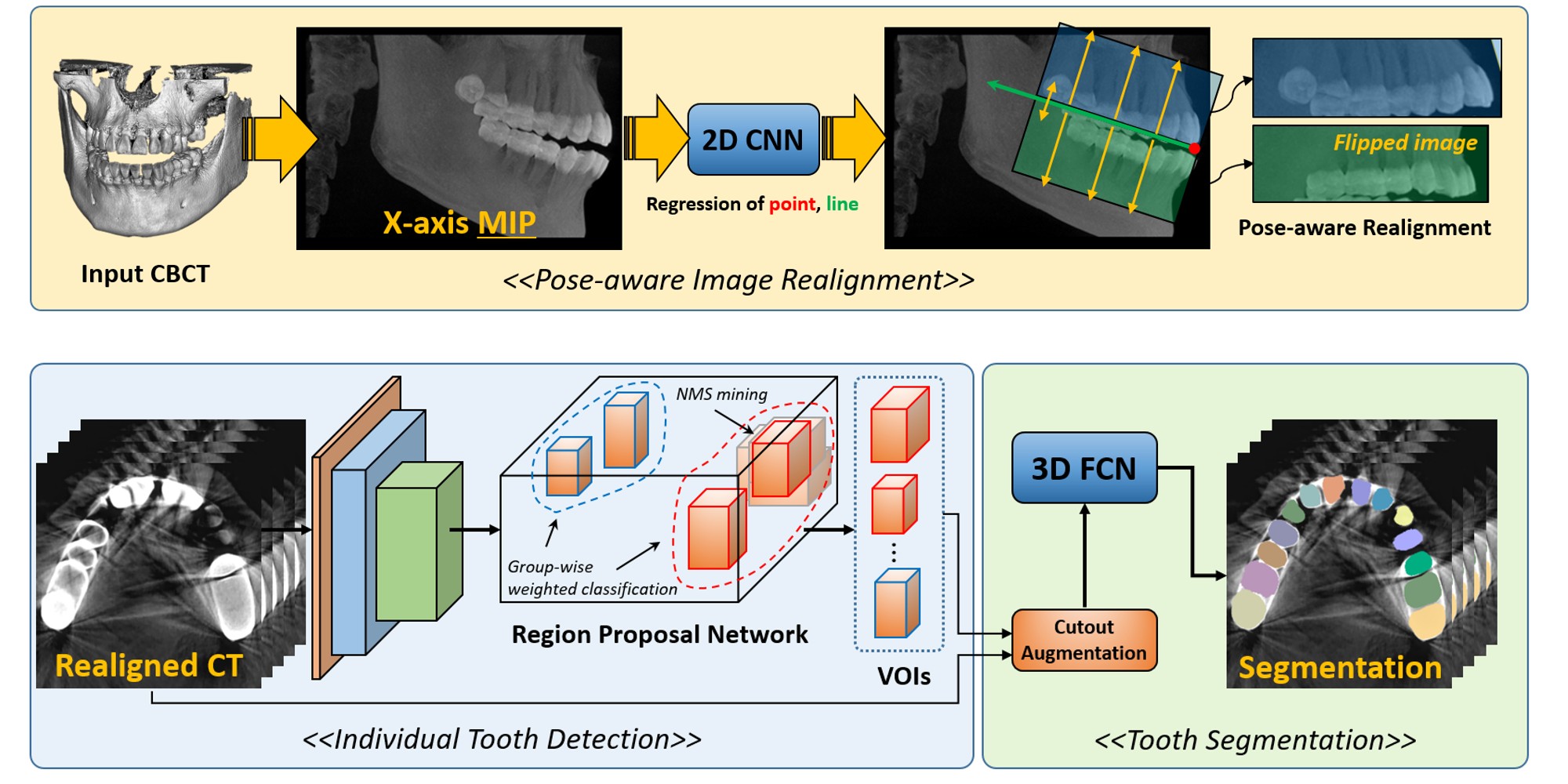 |
Individual tooth segmentation from cone beam computed tomography (CBCT) images is an essential prerequisite for an anatomical understanding of orthodontic structures in several applications, such as tooth reformation planning and implant guide simulations. However, the presence of severe metal artifacts in CBCT images hinders the accurate segmentation of each individual tooth. In this study, we propose a neural network for pixel-wise labeling to exploit an instance segmentation framework that is robust to metal artifacts. Our method comprises of three steps: 1) image cropping and realignment by pose regressions, 2) metal-robust individual tooth detection, and 3) segmentation. We first extract the alignment information of the patient by pose regression neural networks to attain a volume-of-interest (VOI) region and realign the input image, which reduces the inter-overlapping area between tooth bounding boxes. Then, individual tooth regions are localized within a VOI realigned image using a convolutional detector. We improved the accuracy of the detector by employing non-maximum suppression and multiclass classification metrics in the region proposal network. Finally, we apply a convolutional neural network (CNN) to perform individual tooth segmentation by converting the pixel-wise labeling task to a distance regression task. Metal-intensive image augmentation is also employed for a robust segmentation of metal artifacts. The result shows that our proposed method outperforms other state-of-the-art methods, especially for teeth with metal artifacts. Our method demonstrated 5.68% and 30.30% better accuracy in the F1 score and aggregated Jaccard index, respectively, when compared to the best performing state-of-the-art algorithms. The primary significance of the proposed method is two-fold: 1) an introduction of pose-aware VOI realignment followed by a robust tooth detection and 2) a metal-robust CNN framework for accurate tooth segmentation. |
- Hyo Jung Park, Kyoung Won Kim, Jae Hyun Kwon, Jeongjin Lee, Taeyong Park, Heon-Ju Kwon, Gi-Won Song, Sung-Gyu Lee, Lifestyle modification leads to spatially variable reduction in hepatic steatosis in potential live liver donors, Liver Transplantation, Vol. 26, Issue 4, pp. 487-497, April 2020. (doi:10.1002/lt.25733)
- Youngchan Song, Jeongjin Lee, Yeong-Gil Shin, Dongjoon Kim, Confidence Surface-based Fine Matching between Dental CBCT Scan and Optical Surface Scan Data, Journal of Medical Imaging and Health Informatics, Vol. 10, No. 4, pp. 795-806, April 2020. (doi:10.1166/jmihi.2020.2975)
- So Yeong Jeong, Jeongjin Lee, Kyoung Won Kim, Jin Kyoo Jang, Heon-Ju Kwon, Gi Won Song, Sung Gyu Lee, Estimation of the Right Posterior Section Volume in Live Liver Donors: Semi-automated CT Volumetry using Portal Vein Segmentation, Academic Radiology, Vol. 27, Issue 2, pp. 210-218, Feburary 2020. (doi:10.1016/j.acra.2019.03.018)
- Jieun Byun, Kyoung Won Kim, Jeongjin Lee, Heon-Ju Kwon, Jae Hyun Kwon, Gi-Won Song, Sung-Gyu Lee, The role of multiphase CT in patients with acute postoperative bleeding after liver transplantation, Abdominal Radiology, Vol. 45, pp. 141-152, January 2020. (doi:10.1007/s00261-019-02347-y)
- Hyo Jung Park, Yongbin Shin, Hyosang Kim, In Seob Lee, Dong-Woo Seo, Jimi Huh, Tae Young Lee, Taeyong Park, Jeongjin Lee, Kyung Won Kim, Development and Validation of a Deep Learning System for Segmentation of Abdominal Muscle and Fat on Computed Tomography, Korean Journal of Radiology, Vol. 21, No. 1, pp. 88-100, January 2020. (doi:10.3348/kjr.2019.0470)
- Jin Sil Kim, Kyoung Won Kim, Jeongjin Lee, Heon-Ju Kwon, Jae Hyun Kwon, Gi-Won Song, Sung-Gyu Lee, Diagnostic performance for hepatic artery occlusion after liver transplantation: CT angiography vs. contrast-enhanced US, Liver Transplantation, Vol. 25, Issue 11, pp. 1651-1660, November 2019. (doi:10.1002/lt.25588)
- Heon-Ju Kwon, Kyoung Won Kim, Sang Hyun Choi, Jeongjin Lee, Jae Hyun Kwon, Gi-Won Song, Sung-Gyu Lee, Visibility of B1 and R/L Dissociation Using Gd-EOB-DTPA-enhanced T1-weighted MR Cholangiography in Live Liver Transplantation Donors, Transplantation Proceedings, Volume 51, Issue 8, pp. 2735-2739, October 2019. (doi.org/10.1016/j.transproceed.2019.04.085)
- Sunyoung Lee, Kyoung Won Kim, Jeongjin Lee, Taeyong Park, Gi-Won Song, Sung-Gyu Lee, Portal Vein Flow by Doppler Ultrasonography and Liver Volume by Computed Tomography, Experimental and Clinical Transplantation, Volume 17, Issue 5, pp. 627-631, October 2019. (doi:10.6002/ect.2018.0223)
- Taeyong Park, Kyoyeong Koo, Juneseuk Shin, Jeongjin Lee (Corresponding author), Kyung Won Kim, Rapid and Accurate Registration Method between Intra-Operative 2D XA and Pre-operative 3D CTA Images for Guidance of Percutaneous Coronary Intervention, Computational and Mathematical Methods in Medicine, Vol. 2019, pp. 1-12, August 2019. (doi:10.1155/2019/3253605)
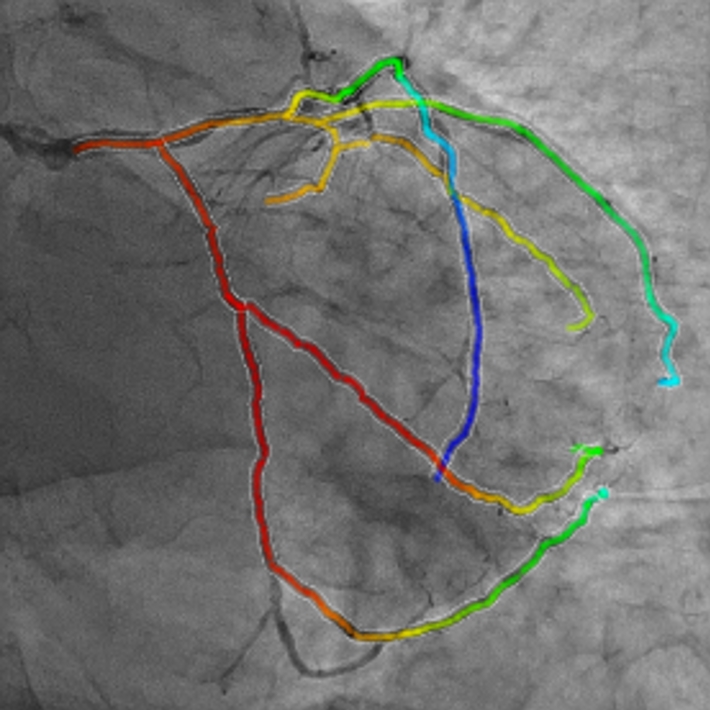 |
In this paper, we propose a rapid rigid registration method for the fusion visualization of intra-operative 2D X-ray angiogram (XA) and pre-operative 3D computed tomography angiography (CTA) images. First, we perform the cardiac cycle alignment of a patient¡¯s 2D XA and 3D CTA images obtained from different apparatus. Subsequently, we perform the initial registration through alignment of the registration space and optimal boundary box. Finally, the two images are registered where the distance between two vascular structures is minimized by using the local distance map, selective distance measure, and optimization of transformation function. To improve the accuracy and robustness of the registration process, the normalized importance value based on the anatomical information of the coronary arteries is utilized. The experimental results showed fast, robust, and accurate registration using 10 cases each of the left coronary artery (LCA) and right coronary artery (RCA). Our method can be used as a computer-aided technology for percutaneous coronary intervention (PCI). Our method can be applied to the study of other types of vessels. |
- Hyo Jung Park, Kyoung Won Kim, Sang Hyun Choi, Jeongjin Lee, Heon-Ju Kwon, Jae Hyun Kwon, Gi-Won Song, Sung-Gyu Lee, Dilatation of left portal vein after right portal vein embolization: a simple estimation for growth of future liver remnant, Journal of Hepato-Biliary-Pancreatic Sciences, Vol. 26, Issue 7, pp. 300-309, July 2019. (doi:10.1002/jhbp.633)
- Taeyong Park, Sunhye Lim, Heeryeol Jeong, Juneseuk Shin, Jeongjin Lee (Corresponding author), Accurate Extraction of Coronary Vascular Structures in 2D X-ray Angiogram using Vascular Topology Information in 3D Computed Tomography Angiography, Journal of Medical Imaging and Health Informatics, Vol. 9, No. 2, pp. 242-250, Feburary 2019. (doi:10.1166/jmihi.2019.2595)
 |
Since the 2D X-ray angiogram enables the detection of vascular stenosis in real-time, it is essential for percutaneous coronary intervention (PCI). However, the accurate vascular structure is very difficult to determine due to background clutter and loss of depth information of 2D projection. To cope with these difficulties, we propose a fast and accurate extraction method of a vascular structure in 2D X-ray angiogram (XA) based on the vascular topology information of 3D computed tomography angiography (CTA) of the same patient. First, an initial vascular structure is robustly extracted based on vessel enhancement filtering. Then, 2D XA and 3D CTA are spatially aligned by 2D-3D registration. Finally, the 2D vascular structure is accurately reconstructed based on 3D vascular topology information by measuring the similarity of 2D and 3D vascular segments. Experimental results showed the fast and accurate extraction of a vascular structure using 10 images each of the left coronary artery (LCA) and right coronary artery (RCA). Our method can be used as a computer-aided technology for PCI. Our method can be applied to the study of other various types of vessels. |
- Jieun Byun, Kyoung Won Kim, Sang Hyun Choi, Sunyoung Lee, Jeongjin Lee, Gi Won Song, Sung Gyu Lee, Indirect Doppler Ultrasound Abnormalities of Significant Portal Vein Stenosis After Liver Transplantation, Journal of Medical Ultrasonics, Vol. 46, Issue. 1, pp. 89-98, January 2019. (doi:10.1007/s10396-018-0894-x)
- Minyoung Chung, Jeongjin Lee (Corresponding author), Jin Wook Chung, Yeong-Gil Shin, Accurate Liver Vessel Segmentation via Active Contour Model with Dense Vessel Candidates, Computer Methods and Programs in Biomedicine, Vol. 166, Issue. 1, pp. 61-75, November 2018. (doi:10.1016/j.cmpb.2018.10.010)
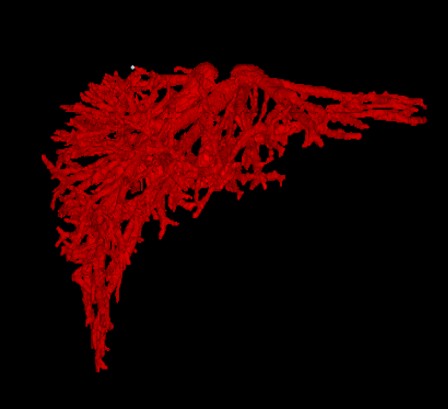 |
Background and Objective: The purpose of this paper is to propose a fully automated liver vessel segmentation algorithm including portal vein and hepatic vein on contrast enhanced CTA images. Methods: First, points of a vessel candidate region are extracted from 3-dimensional (3D) CTA image. To generate accurate points, we reduce 3D segmentation problem to 2D problem by generating multiple maximum intensity (MI) images. After the segmentation of MI images, we back-project pixels to the original 3D domain. We call these voxels as vessel candidates (VCs). A large set of MI images can produce very dense and accurate VCs. Finally, for the accurate segmentation of a vessel region, we propose a newly designed active contour model (ACM) that uses the original image, vessel probability map from dense VCs, and the good prior of an initial contour. Results: We used 55 abdominal CTAs for a parameter study and a quantitative evaluation. We evaluated the performance of the proposed method comparing with other state-of-the-art ACMs for vascular images applied directly to the original data. The result showed that our method successfully segmented vascular structure 25%-122% more accurately than other methods without any extra false positive detection. Conclusion: Our model can generate a smooth and accurate boundary of the vessel object and easily extract thin and weak peripheral branch vessels. The proposed approach can automatically segment a liver vessel without any manual interaction. The detailed result can aid further anatomical studies. |
- Heewon Kye, Se Hee Lee, Jeongjin Lee (Corresponding author), CPU-based real-time maximim intensity projection via fast matrix transposition using parallelization operations with AVX instruction set, Multimedia Tools and Applications, Vol. 77, Issue. 12, pp. 15971-15994, June 2018. (doi:10.1007/s11042-017-5171-2)
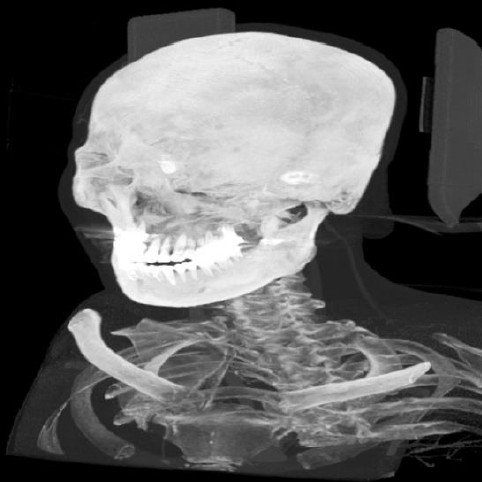 |
Rapid visualization is essential for maximum intensity projection (MIP) rendering, since the acquisition of a perceptual depth can require frequent changes of a viewing direction. In this paper, we propose a CPU-based real-time MIP method that uses parallelization operations with the AVX instruction set. We improve shear-warp based MIP rendering by resolving the bottle-neck problems of the previous method of a matrix transposition. We propose a novel matrix transposition method using the AVX instruction set to minimize bottle-neck problems. Experimental results show that the speed of MIP rendering on general CPU is faster than 20 frame-per-second (fps) for a 512 x 512 x 552 volume dataset. Our matrix transposition method can be applied to other image processing algorithms for faster processing. |
- Heon-Ju Kwon, Kyoung Won Kim, Sang Hyun Choi, Jin-Hee Jung, So Yeon Kim, Se-Young Kim, Jeongjin Lee, Dong-Hwan Jung, Tae-Yong Ha, Gi-Won Song, Sung-Gyu Lee, MR Cholangiography in Potential Liver Donors: Quantitative and Qualitative Improvement with Administration of Oral Effervescent Agent, Journal of Magnetic Resonance Imaging, Vol. 46, No. 6, pp. 1656-1663, December 2017. (doi: 10.1002/jmri.25715)
- Se-Young Kim, Kyoung Won Kim, Sang Hyun Choi, Jae Hyun Kwon, Gi-Won Song, Heon-Ju Kwon, Young Ju Yun, Jeongjin Lee, Sung-Gyu Lee, Feasibility of UltraFast Doppler in postoperative evaluation of hepatic artery in recipients following liver transplantation, Ultrasound in Medicine and Biology, Vol. 43, No. 11, pp. 2611-2618, November 2017. (doi:10.1016/j.ultrasmedbio.2017.07.018)
- Hye Young Jang, Kyoung Won Kim, Jae Hyun Kwon, Heon-Ju Kwon, Bohyun Kim, Nieun Seo, Jeongjin Lee, Gi-Won Song, Sung-Gyu Lee, N-butyl-2 cyanoacrylate (NBCA) embolus in the graft portal vein after portosystemic collateral embolization in liver transplantation recipient: what is the clinical significance?, Acta Radiologica, Vol. 58, Issue 11, pp. 1326-1333, November 2017. (doi:10.1177/0284185117693460 )
- Sang Hyun Choi, Jae Hyun Kwon, Kyoung Won Kim, Hye Young Jang, Ji Hye Kim, Heon-Ju Kwon, Jeongjin Lee, Gi-Won Song, Sung Gyu Lee, Measurement of liver volumes by portal vein flow by Doppler ultrasound in living donor liver transplantation, Clinical Transplantation, Vol. 31, No. 9, pp. 1-9, September 2017. (doi:10.1111/ctr.13050)
- Jeongjin Lee, Changseok Kim, Juneseuk Shin, Technology opportunity discovery to R&D planning: Key technological performance analysis, Technological Forecasting & Social Change, Vol. 119, No. 1, pp. 53-63, June 2017. (doi: 10.1016/j.techfore.2017.03.011)
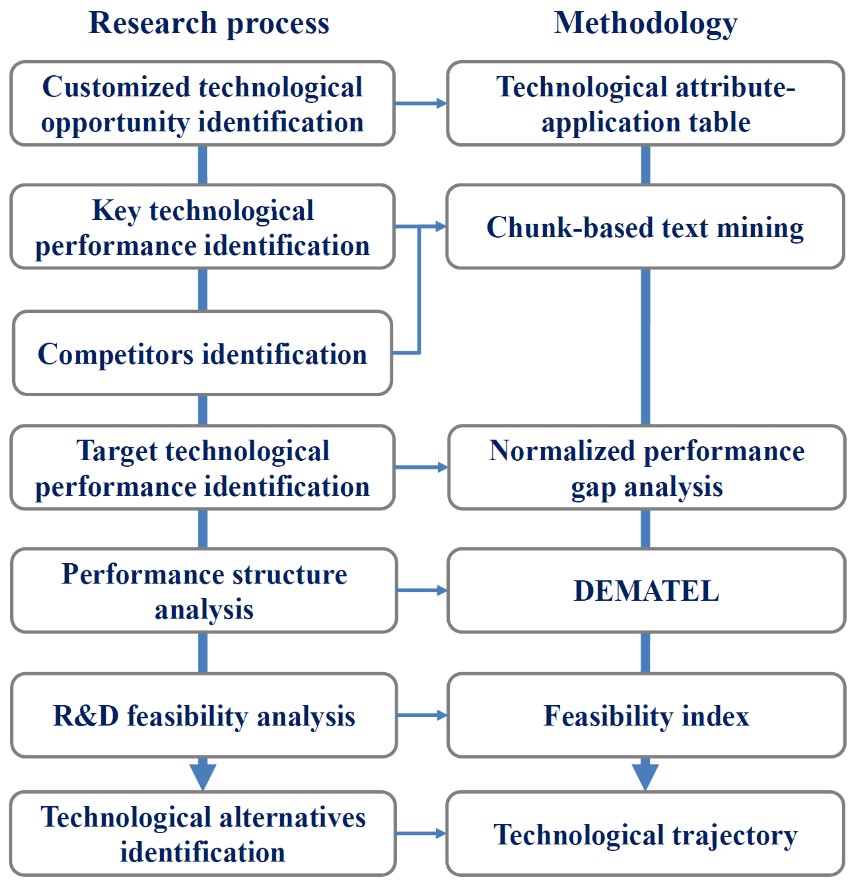 |
There is a gap between technological opportunity identification and R&D planning because opportunity information is not enough to serve needs of R&D planning experts. Addressing this issue, we suggest a method of transforming a broadly defined technological opportunity to a detailed R&D plan. We identify key information for R&D planning, extract such information from bibliometric data by using chunk-based mining, and convert it to an understandable as well as usable form for R&D planning. Dynamic technological performance information of key competitors are collected and used. A systematic analysis of normalized performance gap, performances structure, R&D feasibility and technological alternatives enables R&D experts to identify important and feasible target technological performances and R&D solutions to gain technological advantages. Our method can increase application value of technological opportunities while reducing efforts of experts, thereby making R&D planning more effective as well as efficient. A battery separator opportunity using membrane technology is exemplified. |
- Bohyun Kim, Kyoung Won Kim, So Yeon Kim, So Hyun Park, Jeongjin Lee, Gi Won Song, Dong-Hwan Jung, Tae-Yong Ha, Sung Gyu Lee, Coronal 2D MR cholangiography overestimates the length of the right hepatic duct in liver transplantation donors, European Radiology, Vol. 27, Issue 5, pp. 1822-1830, May 2017. (doi:10.1007/s00330-016-4572-3)
- Ohjae Kwon, Jeongjin Lee (Corresponding author), Bohyoung Kim, Juneseuk Shin, Yeong-Gil Shin, Efficient Blood Flow Visualization using Flowline Extraction and Opacity Modulation based on Vascular Structure Analysis, Computers in Biology and Medicine, Vol. 82, No. 1, pp. 87-99, March 2017. (doi: 10.1016/j.compbiomed.2017.01.020)
 |
With the recent advances regarding the acquisition and simulation of blood flow data, blood flow visualization has been widely used in medical imaging for the diagnosis and treatment of pathological vessels. In this paper, we present a novel method for the visualization of the blood flow in vascular structures. The vessel inlet or outlet is first identified using the orthogonality metric between the normal vectors of the flow velocity and vessel surface. Then, seed points are generated on the identified inlet or outlet by Poisson disk sampling. Therefore, it is possible to achieve the automatic seeding that leads to a consistent and faster flow depiction by skipping the manual location of a seeding plane for the initiation of the line integration. In addition, the early terminated line integration in the thin curved vessels is resolved through the adaptive application of the tracing direction that is based on the flow direction at each seed point. Based on the observation that blood flow usually follows the vessel track, the representative flowline for each branch is defined by the vessel centerline. Then, the flowlines are rendered through an opacity assignment according to the similarity between their shape and the vessel centerline. Therefore, the flowlines that are similar to the vessel centerline are shown transparently, while the different ones are shown opaquely. Accordingly, the opacity modulation method enables the flowlines with an unusual flow pattern to appear more noticeable, while the visual clutter and line occlusion are minimized. Finally, Hue-Saturation-Value color coding is employed for the simultaneous exhibition of flow attributes such as local speed and residence time. The experiment results show that the proposed technique is suitable for the depiction of the blood flow in vascular structures. The proposed approach is applicable to many kinds of tubular structures with embedded flow information. |
- Youngchan Song, Hyunna Lee, Ho Chul Kang, Juneseuk Shin, Gil-Sun Hong, Seong Ho Park, Jeongjin Lee (Corresponding author), Yeong-Gil Shin, Interactive Registration between Supine and Prone Scans in Computed Tomography Colonography using Band-Height Images, Computers in Biology and Medicine, Vol. 80, No. 1, pp. 124-136, January 2017. (doi: 10.1016/j.compbiomed.2016.11.020)
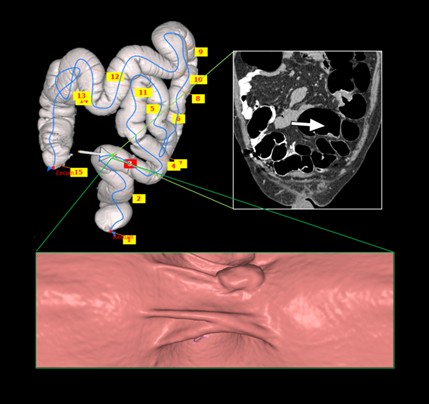 |
In computed tomographic colonography (CTC), a patient is commonly scanned twice including supine and prone scans to improve the sensitivity of polyp detection. Typically, a radiologist must manually match the corresponding areas in the supine and prone CT scans, which is a difficult and time-consuming task, even for experienced scan readers. In this paper, we propose a method of supine-prone registration utilizing band-height images, which are directly constructed from the CT scans using a ray-casting algorithm containing neighboring shape information. In our method, we first identify anatomical feature points and establish initial correspondences using local extreme points on centerlines. We then correct correspondences using band-height images that contain neighboring shape information. We use geometrical and image-based information to match positions between the supine and prone centerlines. Finally, our algorithm searches the correspondence of user input points using the matched anatomical feature point pairs as key points and band-height images. The proposed method achieved accurate matching and relatively faster processing time than other previously proposed methods. The mean error of the matching between the supine and prone points for uniformly sampled positions was 18.41¡¾22.07 mm in 20 CTC datasets. The average pre-processing time was 62.9¡¾8.6 sec, and the interactive matching was performed in nearly real-time. Our supine-prone registration method is expected to be helpful for the accurate and fast diagnosis of polyps. |
- Hyunjoo Song, Jeongjin Lee, Tae Jung Kim, Kyoung Ho Lee, Bohyoung Kim, Jinwook Seo, ¡°GazeDx: Interactive Visual Analytics Framework for Comparative Gaze Analysis with Volumetric Medical Images,¡± IEEE Transactions on Visualization and Computer Graphics, Vol. 23, No. 1, pp. 311-320, January 2017. (doi: 10.1109/TVCG.2016.2598796)
- Jin Sil Kim, Jae Hyun Kwon, Kyoung Won Kim, Jihun Kim, So Yeon Kim, Woo Kyoung Jeong, So Hyun Park, Eunsil Yu, Jeongjin Lee, So Jung Lee, Jong Seok Lee, Hyoung Jung Kim, Gi Won Song, and Sung Gyu Lee, CT Features of Primary Graft Non-function after Liver Transplantation, Radiology, Vol. 281, No. 1, pp. 465-473, November 2016. (doi: 10.1148/radiol.2016152157)
- Jihye Kim, Jeongjin Lee (Corresponding author), Jin Wook Chung, Yeong-Gil Shin, Locally Adaptive 2D-3D Registration using Vascular Structure Model for Liver Catheterization, Computers in Biology and Medicine, Vol. 70, No. 1, pp. 119-130, March 2016. (doi: 10.1016/j.compbiomed.2016.01.009)
 |
Two-dimensional-three-dimensional (2D-3D) registration between intra-operative 2D digital subtraction angiography (DSA) and pre-operative 3D computed tomography angiography (CTA) can be used for roadmapping purposes. However, through the projection of 3D vessels, incorrect intersections and overlaps between vessels are produced because of the complex vascular structure, which make it difficult to obtain the correct solution of 2D?3D registration. To overcome these problems, we propose a registration method that selects a suitable part of a 3D vascular structure for a given DSA image and finds the optimized solution to the partial 3D structure. The proposed algorithm can reduce the registration errors because it restricts the range of the 3D vascular structure for the registration by using only the relevant 3D vessels with the given DSA. To search for the appropriate 3D partial structure, we first construct a tree model of the 3D vascular structure and divide it into several subtrees in accordance with the connectivity. Then, the best matched subtree with the given DSA image is selected using the results from the coarse registration between each subtree and the vessels in the DSA image. Finally, a fine registration is conducted to minimize the difference between the selected subtree and the vessels of the DSA image. In experimental results obtained using 10 clinical datasets, the average distance errors in the case of the proposed method were 2.34 ¡¾ 1.94 mm. The proposed algorithm converges faster and produces more correct results than the conventional method in evaluations on patient datasets. |
- Jihye Yun, Yeo Koon Kim, Eun Ju Chun, Yeong-Gil Shin, Jeongjin Lee (Co-corresponding author), Bohyoung Kim, Stenosis Map for Volume Visualization of Constricted Tubular Structures: Application to Coronary Artery Stenosis, Computer Methods and Programs in Biomedicine, Vol. 124, No. 1, pp. 76-90, Feburary 2016. (doi: 10.1016/j.cmpb.2015.10.019)
 |
Although direct volume rendering (DVR) has become a commodity, effective rendering of interesting features is still a challenge. In one of active DVR application fields, the medicine, radiologists have used DVR for the diagnosis of lesions or diseases that should be visualized distinguishably from other surrounding anatomical structures. One of most frequent and important radiologic tasks is the detection of lesions, usually constrictions, in complex tubular structures. In this paper, we propose a 3D spatial field for the effective visualization of constricted tubular structures, called as a stenosis map which stores the degree of constriction at each voxel. Constrictions within tubular structures are quantified by using newly proposed measures (i.e. line similarity measure and constriction measure) based on the localized structure analysis, and classified with a proposed transfer function mapping the degree of constriction to color and opacity. We show the application results of our method to the visualization of coronary artery stenoses. We present performance evaluations using ten twenty eight clinical datasets, demonstrating high accuracy and efficacy of our proposed method. The ability of our method to saliently visualize the constrictions within tubular structures and interactively adjust the visual appearance of the constrictions proves to deliver a substantial aid in radiologic practice. |
- Yong Geun Lee, Jeongjin Lee, Yeong-Gil Shin, Ho Chul Kang, Low-dose 2D X-ray Angiography Enhancement using 2-axis PCA for the Preservation of Blood-Vessel Region and Noise Minimization, Computer Methods and Programs in Biomedicine, Vol. 123, No. 1, pp. 15-26, January 2016. (doi: 10.1016/j.cmpb.2015.09.011)
- Dong-Joon Kim, Bohyoung Kim, Jeongjin Lee (Corresponding author), Juneseuk Shin, Kyoung Won Kim, Yeong-Gil Shin, High-quality Slab-based Intermixing Method for Fusion Rendering of Multiple Medical Objects, Computer Methods and Programs in Biomedicine, Vol. 123, No. 1, pp. 27-42, January 2016. (doi: 10.1016/j.cmpb.2015.09.009)
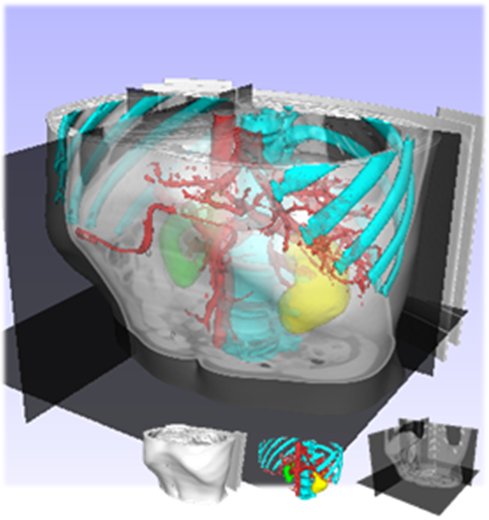 |
The visualization of multiple 3D objects has been increasingly required for recent applications in medical fields. Due to the heterogeneity in data representation or data configuration, it is difficult to efficiently render multiple medical objects in high quality. In this paper, we present a novel intermixing scheme for fusion rendering of multiple medical objects while preserving the real-time performance. First, we present an in-slab visibility interpolation method for the representation of subdivided slabs. Second, we introduce virtual zSlab, which extends an infinitely thin boundary (such as polygonal objects) into a slab with a finite thickness. Finally, based on virtual zSlab and in-slab visibility interpolation, we propose a slab-based visibility intermixing method with the newly proposed rendering pipeline. Experimental results demonstrate that the proposed method delivers more effective multiple-object renderings in terms of rendering quality, compared to conventional approaches. And proposed intermixing scheme provides high-quality intermixing results for the visualization of intersecting and overlapping surfaces by resolving aliasing and z-fighting problems. Moreover, two case studies are presented that apply the proposed method to the real clinical applications. These case studies manifest that the proposed method has the outstanding advantages of the rendering independency and reusability. |
- Ho Chul Kang, Chankyu Choi, Juneseuk Shin, Jeongjin Lee (Corresponding author), Yeong-Gil Shin, Fast and Accurate Semi-automatic Segmentation of Individual Teeth from Dental CT Images, Computational and Mathematical Methods in Medicine, Vol. 2015, pp. 1-12, August 2015. (doi: 10.1155/2015/810796)
 |
Dentists often use three-dimensional CT (Computed Tomography) images for effective implant procedures. During this procedure, the dentists should build individual teeth independently, so the separation of individual teeth is required. However, it is very hard to separate and segment individual teeth automatically, since the brightness of teeth and the brightness of sockets of teeth in dental CT images are very similar. In this paper, we propose a fast and accurate semi-automatic method to effectively distinguish individual teeth from the sockets of teeth in dental CT images. Parameter values of thresholding and shapes of the teeth are propagated to the neighboring slice, based on the separated teeth from reference images. After the propagation of threshold values and shapes of the teeth, the histogram of the current slice was analyzed. The individual teeth are automatically separated and segmented by using seeded region growing. Then, the newly generated separation information is iteratively propagated to the neighboring slice. Our method was validated by ten sets of dental CT scans, and the results were compared with the manually segmented result and conventional methods. The average error of absolute value of volume measurement was 2.29 ¡¾ 0.56%, which was more accurate than conventional methods. Boosting up the speed with the multi-core processors was shown to be 2.4 times faster than a single core processor. The proposed method identified the individual teeth accurately, demonstrating that it can give dentists substantial assistance during dental surgery. |
- Jeongjin Lee, Kyoung Won Kim, So Yeon Kim, Juneseuk Shin, Kyung Jun Park, Hyung Jin Won, Yong Moon Shin, Automatic detection method of hepatocellular carcinomas using the non-rigid registration method of multi-phase liver CT images, Journal of X-Ray Science and Technology, Vol. 23, No. 3, pp. 275-288, May 2015. (doi: 10.3233/XST-150487)
 |
OBJECTIVE: In this paper, we propose the automatic detection method of hepatocellular carcinomas using the non-rigid registration method of multi-phase CT images. METHODS: Global movements between multi-phase CT images are aligned by rigid registration based on normalized mutual information. Local deformations between multi-phase CT images are modeled by non-rigid registration based on B-spline deformable model. After the registration of multi-phase CT images, hepatocellular carcinomas are automatically detected by analyzing the original and subtraction information of the registered multi-phase CT images. RESULTS: We applied our method to twenty five multi-phase CT datasets. Experimental results showed that the multi-phase CT images were accurately aligned. All of the hepatocellular carcinomas including small size ones in our 25 subjects were accurately detected using our method. CONCLUSIONS: We conclude that our method is useful for detecting hepatocellular carcinomas. |
- Youngjoo Lee, Jeongjin Lee (Corresponding author), Binary Tree Optimization using Genetic Algorithm for Multiclass Support Vector Machine, Expert Systems with Applications, Vol. 42, No. 8, pp. 3843-3851, May 2015. (doi: 10.1016/j.eswa.2015.01.022)
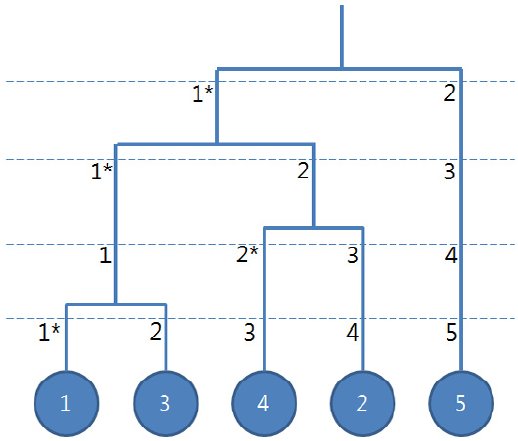 |
In this paper, we propose a global optimization method of a binary tree structure using GA to improve the classification accuracy of multiclass problem for SVM. Unlike previous researches on multiclass SVM using binary tree structures, our approach globally finds the optimal binary tree structure. For the efficient utilization of GA, we propose an enhanced crossover strategy to include the determination method of crossover points and the generation method of offsprings to preserve the maximum information of a parent tree structure. Experimental results showed that the proposed method provided higher accuracy than any other competing methods in 11 out of 18 datasets used as benchmark, within an appropriate time. The performance of our method for small size problems is comparable with other competing methods while more sensible improvements of the classification accuracy are obtained for the medium and large size problems. |
- Chanwoong Jeon, Jeongjin Lee, Juneseuk Shin, Optimal subsidy estimation method using system dynamics and the real option model: Photovoltaic technology case, Applied Energy, Vol. 142, pp. 33-43, 15 March 2015. (doi: 10.1016/j.apenergy.2014.12.067)
- Ho Chul Kang, Bohyoung Kim, Jeongjin Lee (Corresponding author), Juneseuk Shin, Yeong-Gil Shin, Accurate Four-Chamber Segmentation using Gradient-assisted Localized Active Contour Model, Journal of Medical Imaging and Health Informatics, Vol. 5, No. 1, pp. 126-137, February 2015. (doi: 10.1166/jmihi.2015.1368)
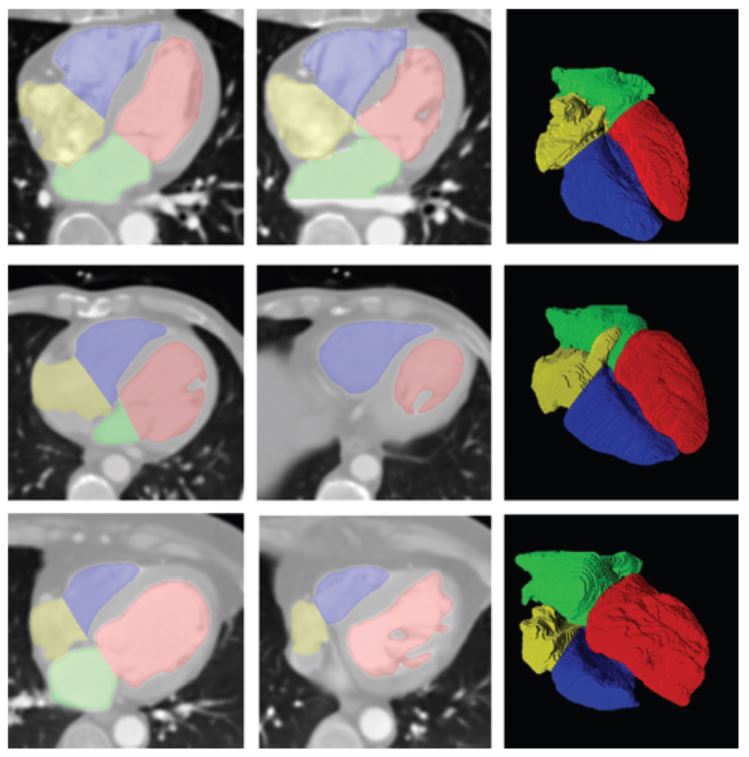 |
In this paper, we propose a novel framework to segment the four chambers of the heart automatically. First, the whole heart is coarsely extracted. This is separated into the left and right parts using a geometric analysis based on anatomical information and a subsequent power watershed. Then, the proposed gradient-assisted localized active contour model (GLACM) refines the left and right sides of the heart segmentation accurately. Our GLACM considers not only region-based information but also edge-based information for a more accurate segmentation compared with a conventional LACM. Finally, the left and right sides of the heart are separated into atrium and ventricle by minimizing the proposed split energy function that determines the boundary between the atrium and ventricle based on the shape and intensity of the heart. In experimental results using twenty clinical datasets, the proposed method identified the four chambers accurately, demonstrating that this approach can assist the cardiologist. |
- Seongjin Park, Ho Chul Kang, Jeongjin Lee (Corresponding author), Juneseuk Shin, Yeong-Gil Shin, An Enhanced Method for Registration of Dental Surfaces Partially Scanned by a 3D Dental Laser Scanning, Computer Methods and Programs in Biomedicine, Vol. 118, No. 1, pp. 11-22, January 2015. (doi: 10.1016/j.cmpb.2014.09.007)
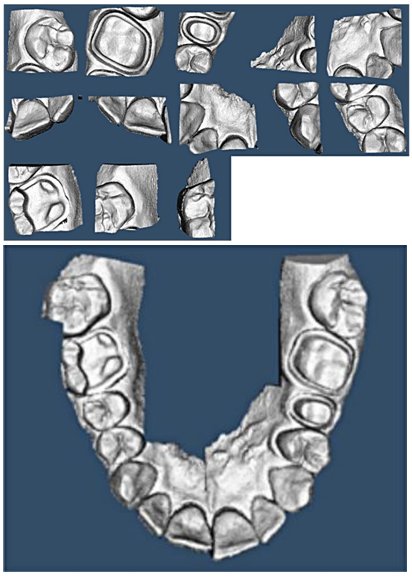 |
In this paper, we propose the fast and accurate registration method of partially scanned dental surfaces in a 3D dental laser scanning. To overcome the multiple point correspondence problems of conventional surface registration methods, we propose the novel depth map-based registration method to register 3D surface models. First, we convert a partially scanned 3D dental surface into a 2D image by generating the 2D depth map image of the surface model by applying a 3D rigid transformation into this model. Then, the image-based registration method using 2D depth map images accurately estimates the initial transformation between two consequently acquired surface models. To further increase the computational efficiency, we decompose the 3D rigid transformation into out-of-plane (i.e. x-, y-rotation, and z-translation) and in-plane (i.e. x-, y-translation, and z-rotation) transformations. For the in-plane transformation, we accelerate the transformation process by transforming the 2D depth map image instead of transforming the 3D surface model. For the more accurate registration of 3D surface models, we enhance iterative closest point (ICP) method for the subsequent fine registration. Our initial depth map-based registration well aligns each surface model. Therefore, our subsequent ICP method can accurately register two surface models since it is highly probable that the closest point pairs are the exact corresponding point pairs. The experimental results demonstrated that our method accurately registered partially scanned dental surfaces. Regarding the computational performance, our method delivered about 1.5 times faster registration than the conventional method. Our method can be successfully applied to the accurate reconstruction of 3D dental objects for orthodontic and prosthodontic treatment. |
- Seongjin Park, Jeongjin Lee, Hyunna Lee, Juneseuk Shin, Jinwook Seo, Kyoung Ho Lee, Yeong-Gil Shin, Bohyoung Kim, Parallelized Seeded Region Growing using CUDA, Computational and Mathematical Methods in Medicine, Vol. 2014, pp. 1-10, September 2014. (doi: 10.1155/2014/856453)
- Jeongjin Lee, Kyoung Won Kim, So Yeon Kim, Bohyoung Kim, So Jung Lee, Hyoung Jung Kim, Jong Seok Lee, Moon Gyu Lee, Gi-Won Song, Shin Hwang, Sung-Gyu Lee, Feasibility of Semi-automated MR Volumetry using Gadoxetic Acid-enhanced MR Images at Hepatobiliary Phase for Living Liver Donors, Magnetic Resonance in Medicine, Vol. 72, No. 3, pp. 640-645, September 2014. (doi: 10.1002/mrm.24964)
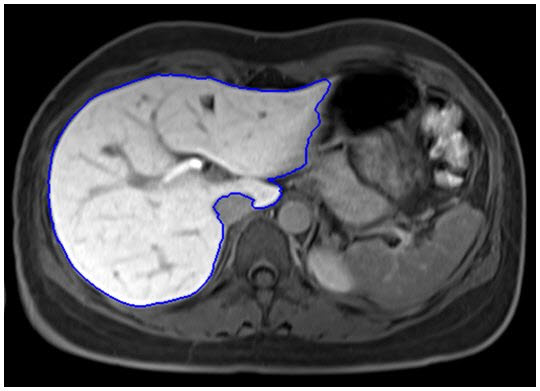 |
PURPOSE: To assess the feasibility of semiautomated MR volumetry using gadoxetic acid-enhanced MRI at the hepatobiliary phase compared with manual CT volumetry. METHODS: Forty potential live liver donor candidates who underwent MR and CT on the same day, were included in our study. Semiautomated MR volumetry was performed using gadoxetic acid-enhanced MRI at the hepatobiliary phase. We performed the quadratic MR image division for correction of the bias field inhomogeneity. With manual CT volumetry as the reference standard, we calculated the average volume measurement error of the semiautomated MR volumetry. We also calculated the mean of the number and time of the manual editing, edited volume, and total processing time. RESULTS: The average volume measurement errors of the semiautomated MR volumetry were 2.35% ¡¾ 1.22%. The average values of the numbers of editing, operation times of manual editing, edited volumes, and total processing time for the semiautomated MR volumetry were 1.9 ¡¾ 0.6, 8.1 ¡¾ 2.7 s, 12.4 ¡¾ 8.8 mL, and 11.7 ¡¾ 2.9 s, respectively. CONCLUSION: Semiautomated liver MR volumetry using hepatobiliary phase gadoxetic acid-enhanced MRI with the quadratic MR image division is a reliable, easy, and fast tool to measure liver volume in potential living liver donors. |
- Hyunna Lee, Jeongjin Lee (Corresponding author), Bohyoung Kim, Se Hyung Kim, Yeong-Gil Shin, Fast Three-Material Modeling with Triple Arch Projection for Electronic Cleansing in CTC, IEEE Transactions on Biomedical Engineering, Vol. 61, No. 7, pp. 2102-2111, July 2014. (doi: 10.1109/TBME.2014.2313888)
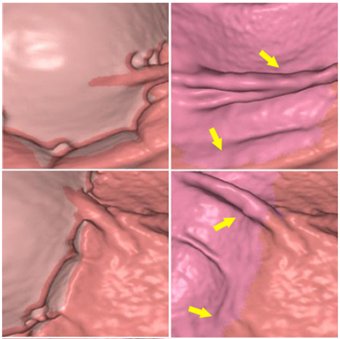 |
In this paper, we propose a fast three-material modeling for electronic cleansing (EC) in computed tomographic colonography. Using a triple arch projection, our three-material modeling provides a very quick estimate of the three-material fractions to remove ridge-shaped artifacts at the T-junctions where air, soft-tissue (ST), and tagged residues (TRs) meet simultaneously. In our approach, colonic components including air, TR, the layer between air and TR, the layer between ST and TR (L(ST/TR)), and the T-junction are first segmented. Subsequently, the material fraction of ST for each voxel in L(ST/TR) and the T-junction is determined. Two-material fractions of the voxels in L(ST/TR) are derived based on a two-material transition model. On the other hand, three-material fractions of the voxels in the T-junction are estimated based on our fast three-material modeling with triple arch projection. Finally, the CT density value of each voxel is updated based on our fold-preserving reconstruction model. Experimental results using ten clinical datasets demonstrate that the proposed three-material modeling successfully removed the T-junction artifacts and clearly reconstructed the whole colon surface while preserving the submerged folds well. Furthermore, compared with the previous three-material transition model, the proposed three-material modeling resulted in about a five-fold increase in speed with the better preservation of submerged folds and the similar level of cleansing quality in T-junction regions. |
- Nam Wook Kim, Jeongjin Lee (Corresponding author), Hyungmin Lee, Jinwook Seo, Accurate Segmentation of Land Regions in Historical Cadastral Maps, Journal of Visual Communication and Image Representation, Vol. 25, No. 5, pp. 1262-1274, July 2014. (doi: 10.1016/j.jvcir.2014.01.001)
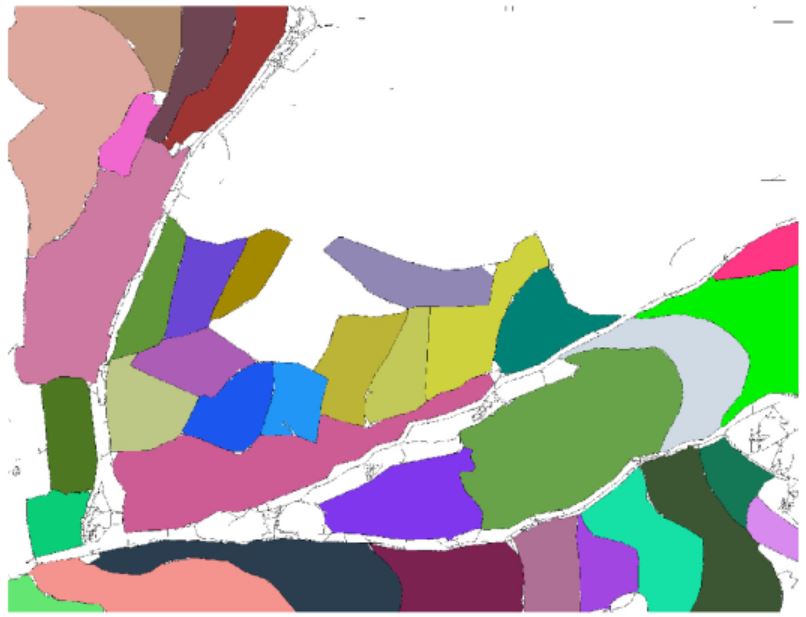 |
In this paper, we propose a novel method of extracting land regions automatically in historical cadastral maps. First, we remove grid reference lines based on the density of the black pixel with the help of the jittering. Then, we remove land owner labels by considering morphological and geometrical characteristics of thinned image. We subsequently reconstruct land boundaries. Finally, the land regions of a user¡¯s interest are modeled by their polygonal approximations. Our segmentation results were compared with manually segmented results and showed that the proposed method extracted the land regions accurately for assisting cadastral mapping in historical research. |
- Sang Hyun Choi, Kyoung Won Kim, So Jung Lee, Jeongjin Lee, So Yeon Kim, Hyoung Jung Kim, Jong Seok Lee, Dong-Hwan Jung, Gi-Won Song, Shin Hwang, Sung-Gyu Lee, Changes in Left Portal Vein Diameter in Live Liver Donors after Right Hemihepatectomy for Living Donor Liver Transplantation, Hepato-gastroenterology, Vol. 61, No. 133, pp. 1380-1386, July 2014.
- Jihyun An, Kyoung Won Kim, S. Han, Jeongjin Lee, Young Suk Lim, Improvement in Survival Associated with Embolisation of Spontaneous Portosystemic Shunt in Patients with Recurrent Hepatic Encephalopathy, Alimentary Pharmacology and Therapeutics, Vol. 39, No. 12, pp. 1418-1426, June 2014. (doi: 10.1111/apt.12771)
- Youngjoo Lee, Jeongjin Lee (Corresponding author), Accurate Automatic Defect Detection Method Using Quadtree Decomposition on SEM Images, IEEE Transactions on Semiconductor Manufacturing, Vol. 27, No. 2, pp. 223-231, May 2014. (doi: 10.1109/TSM.2014.2303473)
- Kyeong-Yeon Nahm, Yong Kim, Yong-Suk Choi, Jeongjin Lee, Seong-Hun Kim, Gerald Nelson, Accurate Registration of the CBCT Scan to the 3D Facial Photograph, American Journal of Orthodontics & Dentofacial Orthopedics, Vol. 145, No. 2, pp. 256-264, Feburary 2014. (doi: 10.1016/j.ajodo.2013.10.018)
- Seongtae Kang, Jeongjin Lee (Corresponding author), Ho Chul Kang, Juneseuk Shin, Yeong-Gil Shin, Feature-preserving Reduction and Visualization of Industrial Volume Data using GLCM Texture Analysis and Mass-spring Model, Journal of Electronic Imaging, Vol. 23, No. 1, pp. 013022-1-013022-10, January 2014. (doi: 10.1117/1.JEI.23.1.013022)
 |
We propose an innovative method that reduces the size of three-dimensional (3-D) volume data while preserving important features in the data. Our method quantifies the importance of features in the 3-D data based on gray level co-occurrence matrix texture analysis and represents the volume data using a simple mass-spring model. According to the measured importance value, blocks containing important features expand while other blocks shrink. After deformation, small features are exaggerated on deformed volume space, and more likely to survive during the uniform volume reduction. Experimental results showed that our method well preserved the small features of the original volume data during the reduction without any artifact compared with the previous methods. Although an additional inverse deformation process was required for the rendering of the deformed volume data, the rendering speed of the deformed volume data was much faster than that of the original volume data. |
- Soon Hyoung Pyo, Jeongjin Lee, Seongjin Park, Yeong-Gil Shin, Kyoung Won Kim, Bohyoung Kim, Physically based Non-rigid Registration using Smoothed Particle Hydrodynamics: Application to Hepatic Metastasis Volume-Preserving Registration, IEEE Transactions on Biomedical Engineering, Vol. 60, No. 9, pp. 2530-2540, September 2013. (doi: 10.1109/TBME.2013.2257172)
- Hyunna Lee, Bohyoung Kim, Jeongjin Lee (Corresponding author), Se Hyung Kim, Yeong-Gil Shin, Tae-Gong Kim, Fold-preserving Electronic Cleansing using a Reconstruction Model Integrating Material Fractions and Structural Responses, IEEE Transactions on Biomedical Engineering, Vol. 60, No. 6, pp. 1546-1555, June 2013. (doi: 10.1109/TBME.2013.2238937)
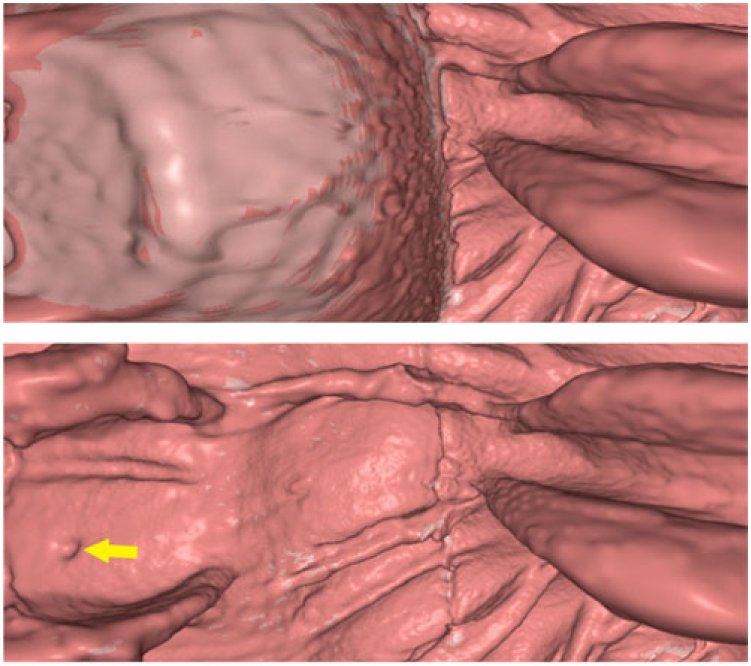 |
In this paper, we propose an electronic cleansing method using a novel reconstruction model for removing tagged materials (TMs) in computed tomography (CT) images. To address the partial volume (PV) and pseudoenhancement (PEH) effects concurrently, material fractions and structural responses are integrated into a single reconstruction model. In our approach, colonic components including air, TM, an interface layer between air and TM, and an interface layer between soft-tissue (ST) and TM (IL ST/TM ) are first segmented. For each voxel in IL ST/TM, the material fractions of ST and TM are derived using a two-material transition model, and the structural response to identify the folds submerged in the TM is calculated by the rut-enhancement function based on the eigenvalue signatures of the Hessian matrix. Then, the CT density value of each voxel in IL ST/TM is reconstructed based on both the material fractions and structural responses. The material fractions remove the aliasing artifacts caused by a PV effect in IL ST/TM effectively while the structural responses avoid the erroneous cleansing of the submerged folds caused by the PEH effect. Experimental results using ten clinical datasets demonstrated that the proposed method showed higher cleansing quality and better preservation of submerged folds than the previous method, which was validated by the higher mean density values and fold preservation rates for manually segmented fold regions. |
- So Jung Lee, Kyoung Won Kim, So Yeon Kim, Yang Shin Park, Jeongjin Lee, Hyoung Jung Kim, Jong Seok Lee, Gi Won Song, Shin Hwang, Sung-Gyu Lee, Contrast-enhanced sonography for screening of vascular complication in recipients following living donor liver transplantation, Journal of Clinical Ultrasound, Vol. 41, No. 5, pp. 305-312, June 2013. (doi: 10.1002/jcu.22044)
- Heon-Ju Kwon, Kyoung Won Kim, So Jung Lee, So Yeon Kim, Jong Seok Lee, Hyoung Jung Kim, Gi-Won Song, Sun A Kim, Eun Sil Yu, Jeongjin Lee, Shin Hwang, Sung Gyu Lee, Value of the Ultrasound Attenuation Index for Noninvasive Quantitative Estimation of Hepatic Steatosis, Journal of Ultrasound in Medicine, Vol. 32, No. 2, pp. 229-235, February 2013.
- Yang Shin Park, Kyoung Won Kim, So Yeon Kim, So Jung Lee, Jeongjin Lee, Jin Hee Kim, Jong Seok Lee, Hyoung Jung Kim, Gi-Won Song, Shin Hwang, Sung-Gyu Lee, Obstruction at Middle Hepatic Venous Tributaries in Modified Right Lobe Grafts after Living-Donor Liver Transplantation: Diagnosis with Contrast-enhanced US, Radiology, Vol. 265, No. 2, pp. 617-626, November 2012. (doi: 10.1148/radiol.12112042)
- Jeongjin Lee, Kyoung Won Kim, Ho Lee, So Jung Lee, Sanghyun Choi, Woo Kyoung Jeong, Hee Won Kye, Gi-Won Song, Shin Hwang, Sung-Gyu Lee, Semiautomated Spleen Volumetry with Diffusion-Weighted MR Imaging, Magnetic Resonance in Medicine, Vol. 68, No. 1, pp. 305-310, July 2012. (doi: 10.1002/mrm.23204)
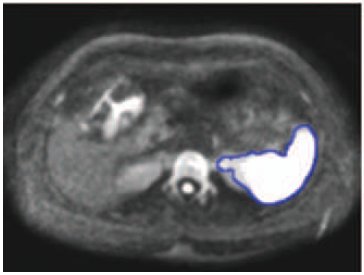 |
In this article, we determined the relative accuracy of semiautomated spleen volumetry with diffusion-weighted (DW) MR images compared to standard manual volumetry with DW-MR or CT images. Semiautomated spleen volumetry using simple thresholding followed by 3D and 2D connected component analysis was performed with DW-MR images. Manual spleen volumetry was performed on DW-MR and CT images. In this study, 35 potential live liver donor candidates were included. Semiautomated volumetry results were highly correlated with manual volumetry results using DW-MR (r = 0.99; P < 0.0001; mean percentage absolute difference, 1.43 ¡¾ 0.94) and CT (r = 0.99; P < 0.0001; 1.76 ¡¾ 1.07). Mean total processing time for semiautomated volumetry was significantly shorter compared to that of manual volumetry with DW-MR (P < 0.0001) and CT (P < 0.0001). In conclusion, semiautomated spleen volumetry with DW-MR images can be performed rapidly and accurately when compared with standard manual volumetry. |
- Heewon Kye, Bong-Soo Sohn, Jeongjin Lee (Corresponding author), Interactive GPU-based Maximum Intensity Projection of Large Medical Data Sets using Visibility Culling based on the Initial Occluder and the Visible Block Classification, Computerized Medical Imaging and Graphics, Vol. 36, No. 5, pp. 366-374, July 2012. (doi: 10.1016/j.compmedimag.2012.04.001)
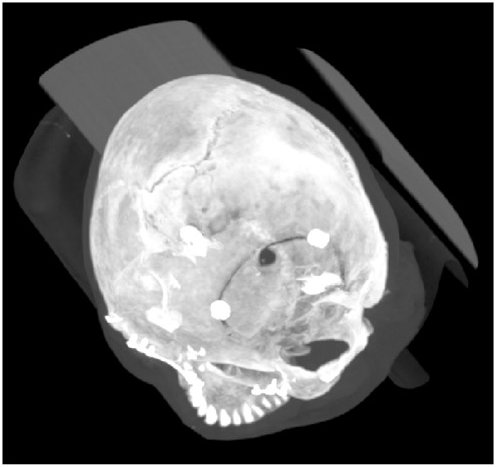 |
In this paper, we propose novel culling methods in both object and image space for interactive MIP rendering of large medical data sets. In object space, for the visibility test of a block, we propose the initial occluder resulting from a preceding image to utilize temporal coherence and increase the block culling ratio a lot. In addition, we propose the hole filling method using the mesh generation and rendering to improve the culling performance during the generation of the initial occluder. In image space, we find out that there is a trade-off between the block culling ratio in object space and the culling efficiency in image space. In this paper, we classify the visible blocks into two types by their visibility. And we propose a balanced culling method by applying a different culling algorithm in image space for each type to utilize the trade-off and improve the rendering speed. Experimental results on twenty CT data sets showed that our method achieved 3.85 times speed up in average without any loss of image quality comparing with conventional bricking method. Using our visibility culling method, we achieved interactive GPU-based MIP rendering of large medical data sets. |
- Kyoung Won Kim, Jeong Kon Kim, Hyuck Jae Choi, Mi-hyun Kim, Jeongjin Lee, Kyoung-Sik Cho, Sonography of the Adrenal Glands in the Adult, Journal of Clinical Ultrasound, Vol. 40, No. 6, pp. 357-363, July 2012. (doi: 10.1002/jcu.21947)
- So Jung Lee, Kyoung Won Kim, Jin Hee Kim, So Yeon Kim, Jong Seok Lee, Hyoung Jung Kim, Dong-Hwan Jung, Gi-Won Song, Shin Hwang, Eun Sil Yu, Jeongjin Lee, Sung-Gyu Lee, Doppler sonography of patients with and without acute cellular rejection after right-lobe living donor liver transplantation, Journal of Ultrasound in Medicine, Vol. 31, No. 6, pp. 845-851, June 2012.
- Gyehyun Kim, Jeongjin Lee (Co-first author), Jinwook Seo, Wooshik Lee, Yeong-Gil Shin, Bohyoung Kim, Automatic Teeth Axes Calculation for Well-Aligned Teeth using Cost Profile Analysis along Teeth Center Arch, IEEE Transactions on Biomedical Engineering, Vol. 59, Issue 4, pp. 1145-1154, April 2012. (doi: 10.1109/TBME.2012.2185825)
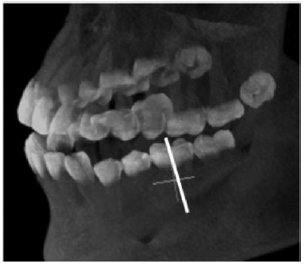 |
This paper presents a novel method of automatically calculating individual teeth axes. The planes separating the individual teeth are automatically calculated using cost profile analysis along the teeth center arch. In this calculation, a novel plane cost function, which considers the intensity and the gradient, is proposed to favor the teeth separation planes crossing the teeth interstice and suppress the possible inappropriately detected separation planes crossing the soft pulp. The soft pulp and dentine of each individually separated tooth are then segmented by a fast marching method with two newly proposed speed functions considering their own specific anatomical characteristics. The axis of each tooth is finally calculated using principal component analysis on the segmented soft pulp and dentine. In experimental results using 20 clinical datasets, the average angle and minimum distance differences between the teeth axes manually specified by two dentists and automatically calculated by the proposed method were 1.94¡Æ ¡¾ 0.61¡Æ and 1.13 ¡¾ 0.56 mm, respectively. The proposed method identified the individual teeth axes accurately, demonstrating that it can give dentists substantial assistance during dental surgery such as dental implant placement and orthognathic surgery. |
- K J LIM, Kyoung Won Kim, Woo Kyoung Jeong, S Y KIM, Yun Jin Jang, S YANG, Jeongjin Lee, Colour Doppler sonography of hepatic haemangiomas with arterioportal shunts, British Journal of Radiology, Vol. 85, No. 1010, pp. 142-146, February 2012. (doi:10.1259/bjr/96605786)
- Seongjin Park, Bohyoung Kim, Jeongjin Lee (Corresponding author), Jin Mo Goo, Yeong-Gil Shin, GGO Nodule Volume-Preserving Nonrigid Lung Registration using GLCM Texture Analysis, IEEE Transactions on Biomedical Engineering, Vol. 58, Issue 10, pp. 2885-2894, October 2011. (doi:10.1109/TBME.2011.2162330)
 |
In this paper, we propose an accurate and fast nonrigid registration method. It applies the volume-preserving constraint to candidate regions of GGO nodules, which are automatically detected by gray-level cooccurrence matrix (GLCM) texture analysis. Considering that GGO nodules can be characterized by their inner inhomogeneity and high intensity, we identify the candidate regions of GGO nodules based on the homogeneity values calculated by the GLCM and the intensity values. Furthermore, we accelerate our nonrigid registration by using Compute Unified Device Architecture (CUDA). In the nonrigid registration process, the computationally expensive procedures of the floating-image transformation and the cost-function calculation are accelerated by using CUDA. The experimental results demonstrated that our method almost perfectly preserves the volume of GGO nodules in the floating image as well as effectively aligns the lung between the reference and floating images. Regarding the computational performance, our CUDA-based method delivers about 20¡¿ faster registration than the conventional method. Our method can be successfully applied to a GGO nodule follow-up study and can be extended to the volume-preserving registration and subtraction of specific diseases in other organs (e.g., liver cancer). |
- Yang Shin Park, Kyoung Won Kim, So Jung Lee, Jeongjin Lee, Dong-Hwan Jung, Gi-Won Song, Tae-Yong Ha, Deok-Bog Moon, Ki-Hun Kim, Chul-Soo Ahn, Shin Hwang, Sung-Gyu Lee, Hepatic Arterial Stenosis Assessed with Doppler US after Liver Transplantation: Frequent False-Positive Diagnoses with Tardus Parvus Waveform and Value of Adding Optimal Peak Systolic Velocity Cutoff, Radiology, Vol. 260, No. 3, September 2011. (doi: 10.1148/radiol.11102257)
- J.H. Kim, K.W. Kim, D.I. Gwon, G.Y. Ko, K.B. Sung, J. Lee, Y.M. Shin, G.W. Song, S. Hwang, S.G. Lee, Effect of splenic artery embolization for splenic artery steal syndrome in liver transplant recipients: estimation at computed tomography based on changes in caliber of related arteries, Transplantation Proceedings, Vol. 43, No. 5, pp. 1790-1793, 2011. (doi:10.1016/j.transproceed.2011.02.022)
- Sang Ok Park, Joon Beom Seo, Namkug Kim, Young Kyung Lee, Jeongjin Lee, Dong Soon Kim, Comparison of Usual Interstitial Pneumonia and Nonspecific Interstitial Pneumonia: Quantification of Disease Severity and Discrimination between Two Diseases on HRCT Using a Texture-Based Automated System, Korean Journal of Radiology, Vol. 12, No. 3, pp. 297-307, 2011.
- Gyehyun Kim, Jeongjin Lee, Ho Lee, Jinwook Seo, Yun-Mo Koo, Yeong-Gil Shin, Bohyoung Kim, Automatic extraction of inferior alveolar nerve canal using feature-enhancing panoramic volume rendering, IEEE Transactions on Biomedical Engineering, Vol. 58, No. 2, pp. 253-264, Feburary 2011. (doi: 10.1109/TBME.2010.2089053)
- Hyun Woo Goo, Dong Hyun Yang, Soo-Jong Hong, Jinho Yu, Byoung-Ju Kim, Joon Beom Seo, Eun Jin Chae, Jeongjin Lee, Bernhard Krauss, Xenon ventilation CT using dual-source and dual-energy technique in children with bronchiolitis obliterans: correlation of xenon and CT density values with pulmonary function test results, Pediatric Radiology, Vol. 40, No. 9, pp. 1490-1497, September 2010. (doi: 10.1007/s00247-010-1645-3)
- Kyoung Won Kim, Jeongjin Lee, Ho Lee, Woo Kyoung Jeong, Hyung Jin Won, Yong Moon Shin, Dong-Hwan Jung, Jeong Ik Park, Gi-Won Song, Tae-Yong Ha, Deok-Bog Moon, Ki-Hun Kim, Chul-Soo Ahn, Shin Hwang, Sung-Gyu Lee, Right Lobe Estimated Blood-free Weight for Living Donor Liver Transplantation: Accuracy of Automated Blood-free CT Volumetry-Preliminary Results 1, Radiology, Vol. 256, No. 2, pp. 433-440, August 2010. (doi: 10.1148/radiol.10091897)
- Ho Lee, Jeongjin Lee, Yeong Gil Shin, Rena Lee, Lei Xing, Fast and accurate marker-based projective registration method for uncalibrated transmission electron microscope tilt series, Physics in Medicine and Biology, Vol. 55, No. 12, pp. 3417-3440, June 2010. (doi:10.1088/0031-9155/55/12/010)
- Eun Jin Chae, Joon Beom Seo, Jeongjin Lee, Namkug Kim, Hyun Woo Goo, Hyun Joo Lee, Choong Wook Lee, Seung Won Ra, Yeon-Mok Oh, You Sook Cho, Xenon Ventilation Imaging Using Dual-Energy Computed Tomography in Asthmatics: Initial Experience, Investigative Radiology, Vol. 45, No. 6, pp. 354-361, June 2010. (doi: 10.1097/RLI.0b013e3181dfdae0)
- Ho Lee, Jeongjin Lee, Namkug Kim, In Kyoon Lyoo, Yeong Gil Shin, Robust and fast shell registration in PET and MR/CT brain images, Computers in Biology and Medicine, Vol. 39, No. 11, pp. 961-977, November 2009. (doi:10.1016/j.compbiomed.2009.07.009)
- Sang Ok Park, Joon Beom Seo, Namkug Kim, Seong Hoon Park, Young Kyung Lee, Bum-Woo Park, Yu Sub Sung, Youngjoo Lee, Jeongjin Lee, Suk-Ho Kang, Feasibility of automated quantification of regional disease patterns seen with high-resolution computed tomography of patients with various diffuse lung diseases, Korean Journal of Radiology, Vol. 10, No. 5, pp. 455-463, September 2009. (doi: 10.3348/kjr.2009.10.5.455)
- Taek-Hee Lee, Jeongjin Lee (Corresponding author), Ho Lee, Heewon Kye, Yeong Gil Shin, Soo-Hong Kim, Fast perspective volume ray casting method using GPU-based acceleration techniques for translucency rendering in 3D endoluminal CT colonography, Computers in Biology and Medicine, Vol. 39, No. 8, pp. 657-666, August 2009. (doi:10.1016/j.compbiomed.2009.04.007)
 |
In this paper, we propose an efficient GPU-based acceleration technique of fast perspective volume ray casting for translucency rendering in computed tomography (CT) colonography. The empty space searching step is separated from the shading and compositing steps, and they are divided into separate processing passes in the GPU. Using this multi-pass acceleration, empty space leaping is performed exactly at the voxel level rather than at the block level, so that the efficiency of empty space leaping is maximized for colon data set, which has many curved or narrow regions. In addition, the numbers of shading and compositing steps are fixed, and additional empty space leapings between colon walls are performed to increase computational efficiency further near the haustral folds. Experiments were performed to illustrate the efficiency of the proposed scheme compared with the conventional GPU-based method, which has been known to be the fastest algorithm. The experimental results showed that the rendering speed of our method was 7.72fps for translucency rendering of 1024x1024 colonoscopy image, which was about 3.54 times faster than that of the conventional method. Since our method performed the fully optimized empty space leaping for any kind of colon inner shapes, the frame-rate variations of our method were about two times smaller than that of the conventional method to guarantee smooth navigation. The proposed method could be successfully applied to help diagnose colon cancer using translucency rendering in virtual colonoscopy. |
- Tobias Heimann, Bram van Ginneken, Martin Styner, Yulia Arzhaeva, Volker Aurich, Christian Bauer, Andreas Beck, Christoph Becker, Reinhard Beichel, Gyorgy Bekes, Fernando Bello, Gerd Binnig, Horst Bischof, Alexander Bornik, Peter M. M. Cashman, Ying Chi, Andres Cordova, Benoit M. Dawant, Marta Fidrich, Jacob Furst, Daisuke Furukawa, Lars Grenacher, Joachim Hornegger, Dagmar Kainmuller, Richard I. Kitney, Hidefumi Kobatake, Hans Lamecker, Thomas Lange, Jeongjin Lee, Brian Lennon, Rui Li, Senhu Li, Hans-Peter Meinzer, Gabor Nemeth, Daniela S. Raicu, Anne-Mareike Rau, Eva van Rikxoort, Mikael Rousson, Laszlo Rusko, Kinda A. Saddi, Gunter Schmidt, Dieter Seghers, Akinobu Shimizu, Pieter Slagmolen, Erich Sorantin, Grzegorz Soza, Ruchaneewan Susomboon, Jonathan M. Waite, Andreas Wimmer, Ivo Wolf, Comparison and evaluation of methods for liver segmentation from CT datasets, IEEE Transactions on Medical Imaging, Vol. 28, No. 8, pp. 1251-1265, August 2009. (doi: 10.1109/TMI.2009.2013851)
- Seung Soo Lee, Seong Ho Park, Jin Kook Kim, Namkug Kim, Jeongjin Lee, Beom Jin Park, Young Jun Kim, Min Woo Lee, Ah Young Kim, Hyun Kwon Ha, Panoramic endoluminal display with minimal image distortion using circumferential radial ray-casting for primary three-dimensional interpretation of CT colonography, European Radiology, Vol. 19, No. 8, pp. 1951-1959, August 2009. (doi: 10.1007/s00330-009-1362-1)
- Hyunkyung Yoo, Gi-Young Ko, Dong Il Gwon, Jin-Hyoung Kim, Hyun-Ki Yoon, Kyu-Bo Sung, Namguk Kim, Jeongjin Lee, Preoperative portal vein embolization using an amplatzer vascular plug, European Radiology, Vol. 19, No. 5, pp. 1054-1061, May 2009. (doi: 10.1007/s00330-008-1240-2)
- Jeongjin Lee, Gyehyun Kim, Ho Lee, Byeong-Seok Shin, Yeong Gil Shin, Fast path planning in virtual colonoscopy, Computers in Biology and Medicine, Vol. 38, No. 9, pp. 1012-1023, September 2008. (doi: 10.1016/j.compbiomed.2008.07.002)
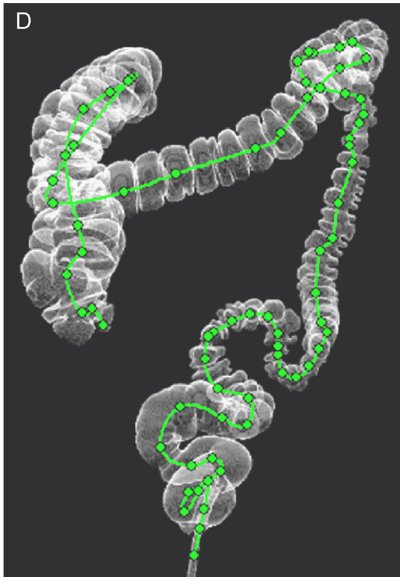 |
We propose a fast path planning algorithm using multi-resolution path tree propagation and farthest visible point. Initial path points are robustly generated by propagating the path tree, and all internal voxels locally most distant from the colon boundary are connected. The multi-resolution scheme is adopted to increase computational efficiency. Control points representing the navigational path are successively selected from the initial path points by using the farthest visible point. The position of the initial path point in a down-sampled volume is accurately adjusted in the original volume. Using the farthest visible point, the number of control points is adaptively changed according to the curvature of the colon shape so that more control points are assigned to highly curved regions. Furthermore, a smoothing step is unnecessary since our method generates a set of control points to be interpolated with the cubic spline interpolation. We applied our method to 10 computed tomography datasets. Experimental results showed that the path was generated much faster than using conventional methods without sacrificing accuracy, and clinical efficiency. The average processing time was approximately 1s when down-sampling by a factor of 2, 3, or 4. We concluded that our method is useful in diagnosing colon cancer using virtual colonoscopy. |
- Ho Lee, Jeongjin Lee, Namkug Kim, Sang Joon Kim, Yeong Gil Shin, Robust feature-based registration using a Gaussian-weighted distance map and brain feature points for brain PET/CT images, Computers in Biology and Medicine, Vol. 38, No. 9, pp. 945-961, September 2008. (doi: 10.1016/j.compbiomed.2008.04.001)
- Helen Hong, Jeongjin Lee (Corresponding author), Yeny Yim, Automatic lung nodule matching on sequential CT images, Computers in Biology and Medicine, Vol. 38, No. 5, pp. 623-634, May 2008. (doi:10.1016/j.compbiomed.2008.02.010)
 |
We propose an automatic segmentation and registration method that provides more efficient and robust matching of lung nodules in sequential chest computed tomography (CT) images. Our method consists of four steps. First, the lungs are extracted from chest CT images by the automatic segmentation method. Second, gross translational mismatch is corrected by optimal cube registration. This initial alignment does not require extracting any anatomical landmarks. Third, the initial alignment is step-by-step refined by hierarchical surface registration. To evaluate the distance measures between lung boundary points, a three-dimensional distance map is generated by narrow-band distance propagation, which drives fast and robust convergence to the optimal value. Finally, correspondences of manually detected nodules are established from the pairs with the smallest Euclidean distances. Experimental results show that our segmentation method accurately extracts lung boundaries and the registration method effectively finds the nodule correspondences. |
- Jeongjin Lee, Namkug Kim, Ho Lee, Joon Beom Seo, Hyung Jin Won, Yong Moon Shin, Yeong Gil Shin, Soo-Hong Kim, Efficient liver segmentation using a level-set method with optimal detection of the initial liver boundary from level-set speed images, Computer Methods and Programs in Biomedicine, Vol. 88, No. 1, pp. 26-38, October 2007. (doi: 10.1016/j.cmpb.2007.07.005)
 |
In this study, we propose a fast and accurate liver segmentation method from contrast-enhanced computed tomography (CT) images. We apply the two-step seeded region growing (SRG) onto level-set speed images to define an approximate initial liver boundary. The first SRG efficiently divides a CT image into a set of discrete objects based on the gradient information and connectivity. The second SRG detects the objects belonging to the liver based on a 2.5-dimensional shape propagation, which models the segmented liver boundary of the slice immediately above or below the current slice by points being narrow-band, or local maxima of distance from the boundary. With such optimal estimation of the initial liver boundary, our method decreases the computation time by minimizing level-set propagation, which converges at the optimal position within a fixed iteration number. We utilize level-set speed images that have been generally used for level-set propagation to detect the initial liver boundary with the additional help of computationally inexpensive steps, which improves computational efficiency. Finally, a rolling ball algorithm is applied to refine the liver boundary more accurately. Our method was validated on 20 sets of abdominal CT scans and the results were compared with the manually segmented result. The average absolute volume error was 1.25+/-0.70%. The average processing time for segmenting one slice was 3.35 s, which is over 15 times faster than manual segmentation or the previously proposed technique. Our method could be used for liver transplantation planning, which requires a fast and accurate measurement of liver volume. |
- Moon Koo Kang, Jeongjin Lee, A real-time cloth draping simulation algorithm using conjugate harmonic functions, Computer & Graphics, Vol. 31, No. 2, pp. 271-279, April 2007. (doi: 10.1016/j.cag.2006.09.010)
|